From Data to Knowledge Management: What to Consider

Although data and knowledge are both stand-alone disciplines that need to be systematically managed, they also must have a connection. Understanding the relationship between data and knowledge management processes and how people are leveraging advances like Pharma 4.0™ combined with these processes enables quality data transition to knowledge that can help pharmaceutical companies. The authors also want to generate understanding on how using the knowledge acquired by people through experience (tacit knowledge) can further connect both data and knowledge management systems, yield positive strategic results, and deliver more efficient processes within organizations.
Knowledge management (KM) is a stand-alone discipline; however, it has relationships with other disciplines. This article explores the relationship between data and knowledge, a deeper look that follows up on the Pharmaceutical Knowledge Ecosystem,
The origin of this ecosystem builds upon the data, information, knowledge, and wisdom (DIKW) hierarchy.
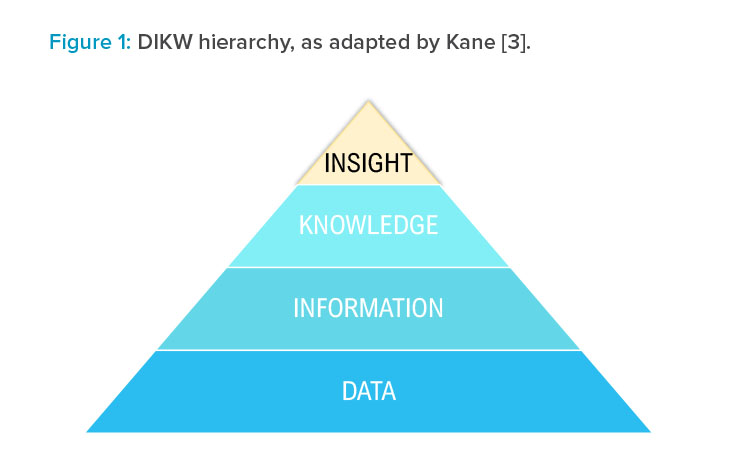
Kane reported that wisdom is widely agreed to be a “uniquely human” characteristic, whereas insights take into account current technological advances and allow data transformation to lead to insights. Although insights may be derived by people with knowledge and experience, they may also be derived from computing or machine-learning models that identify trends and correlations previously not possible to see from experience alone.
Following on from that: Although it is useful to replace wisdom with insights in the DIKW hierarchy, on reflection, Lipa
Mastering the progression of data to information to knowledge to insights and understanding (DIKIU) presents the opportunity to be able to make informed and effective decisions based on accumulated evidence, as provided by the underlying structure.
Data versus Knowledge
In everyday conversations, it is not unusual to hear the words data and knowledge used interchangeably. This section offers definitions and descriptions of these terms.
The Cambridge Dictionary defines data as “information, especially facts or numbers, collected to be examined and considered and used to help decision making, or information in an electronic form that can be stored and used by a computer.”
The definition of data emphasizes information in its raw form, without context. It is context and understanding that increases data’s usefulness and transforms it into knowledge.
From the definitions of data and knowledge, it is clear that having information or understanding about a subject is gained through experience. It should be noted that experience is known or gained by people.
Managing Data and Knowledge
Managing Data
Transferring data to knowledge does not typically happen organically. Procedures that enable users to derive value (e.g., lead to decision-making or in-sights) from an organization’s data or knowledge base should be in place to ensure the information can be validated and trusted. To do this, there should be several procedures in place.
The ISPE GAMP RDI Good Practice Guide: Data Integrity by Design has described managing data as a life-cycle process with five phases.
- Creation
- Processing
- Review, reporting, and use
- Retention and retrieval
- Destruction
The authors of this article would like to highlight and include two further important activities and processes for managing data to this list within Table 1: data governance and data integrity.
Table 1 highlights examples of data-related processes and why they are important.
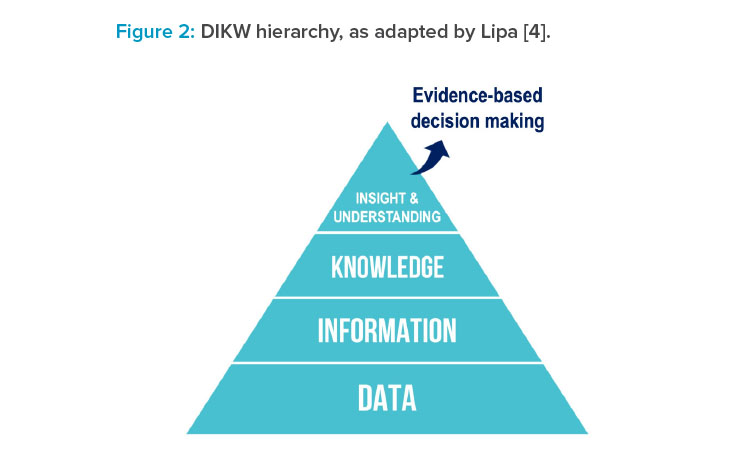
Process | Reason for Importance |
---|---|
Data governance | Governance refers to what decisions must be made to ensure effective management and use of IT (decision domains) and who makes the decisions (locus of accountability for decision-making). |
Creation: data creation and collection |
Many different data sources exist; generally the use of
|
Processing: data analysis and processing |
The main purpose of collecting and analyzing data in commercial manufacturing is to set up a product and process control environment. Raw data is given context by adding information and explaining what the data means, thus presenting information in a required format. |
Retention and retrieval: data retention and retrieval |
In routine manufacturing, manufacturing execution systems
|
Review, reporting, and use: data storage, dissemination, reporting, and use |
Once generated, the data and information require long-term storage and simple reuse options. KM tools organize the acquisition, storage, and dissemination of the product knowledge. |
Destruction: data destruction |
Ensure the correct original data is disposed of after the required retention period. |
Data integrity | Product data should ensure end-to-end traceability and data integrity in order to release a batch. It is expected that the integrity of pharmaceutical data assets should be compliant with attributable, legible, contemporaneous, original, and accurate (ALCOA) principles. |
Managing Knowledge
As with other management disciplines, definitions for KM are plentiful. In this article and in alignment with pharmaceutical industry related literature, two definitions are highlighted:
KM processes can assist in ensuring knowledge is shared in the form it is required for the end user and it is communicated, consistent, and findable
ICH Q10 defines KM as:
A systematic approach to acquiring, analysing, storing and disseminating information related to products, manufacturing processes and components. Sources of knowledge include but are not limited to prior knowledge (public domain or internally documented); pharmaceutical development studies; technology transfer activities; process validation studies over the product lifecycle; manufacturing experience; innovation; continual improvement; and change management activities.
American Productivity and Quality Center (APQC) defines KM as:
The application of a structured process to help information and knowledge flow to the right people at the right time so they can act more efficiently and effectively to find, understand, share, and use knowledge to create value.
The ICH definition describes KM with a more narrow perspective than the APQC definition; the APQC definition is more commonly used by KM practitioners because it embraces the two main aspects of KM: The needs of the knowledge user and the needs of managing knowledge within an organization.
Table 2 presents examples of KM processes and tools that enable a systematic approach to knowledge flow and indicating their importance. These KM are discussed in length in the ISPE Good Practice Guide: Knowledge Management in the Pharmaceutical Industry.
Relationship between Data and Knowledge
Some challenges in assessing the relationship between data and knowledge include large volumes of information make it difficult to focus on the most important elements; multigenerational preferences in the workplace for consuming information; the concept of data privacy; and demonstration of the KM value proposition, which enables buy-in and sponsorship, embedding the concept of knowledge as an asset.
Processes and/or Tools |
Reason for Importance |
KM plan KM maturity assessment |
These are required for planning, understanding requirements of the organization, and defining the process. |
Content management Searching platforms Product knowledge |
These relate mostly to explicit-based knowledge: “a declarative type of knowledge that can be readily articulated (in words or images), coded, stored, and accessed.” |
Communities of Practice Lessons learned Tacit knowledge retention |
These relate mostly to tacit knowledge: “a context-specific type of knowledge, acquired through personal experience or internalization and would reside within people’s minds rather than a physical media or information system. Often referred to as ‘know how.’” Tacit knowledge is gained through experience. It is rarely written down and is hard to capture and validate, but when applied, it increases right first time (RFT) and facilitates continual improvement. |
KM roles KM training KM governance |
Enablers to the KM process. |
It is through data analysis and processing that the relationship between data and knowledge becomes evident. To manage the large volumes of information and extract the important elements, the analysis and processing of data has to add value. To focus on what that value is for an organization, define the objective that an organization or a team needs to achieve from the data, perhaps in the format of a problem statement. To solve the problem, one needs to understand what sources of data and information are needed, and in particular what type of analysis is to be carried out. For example:
- Descriptive analysis: Identifies what has already happened.
- Diagnostic analysis: Focuses on understanding why something happened.
- Predictive analysis: Allows one to identify future trends based on historical data.
- Prescriptive analysis: Allows one to make recommendations for the future.
After the sources of data and information needed are identified and the type of analysis determined, the required data should be collected and aggregated. This includes quantitative (numerical) data or qualitative (descriptive) data. In the pharmaceutical sector, several types of data management platforms that automate data collection are used; some examples can be found in Table 1.
The data from these platforms can be considered “clean” (i.e., data that has had errors, duplicates, and unwanted data points removed) because they are validated systems. The data is reported in a structured manner.
It is through the analysis of data that information, knowledge, and insights are gained. These insights should be shared within the organization with key members who need them. This flow of knowledge is important because raw data will yield no value without knowledge; thus, analysis is needed, which enables insights to be shared in a digestible manner by everyone who receives the information.
Often key decisions are made based on these insights, which have been communicated in the form of reports, dashboards, and interactive visualizations, so they must be clear and unambiguous. Ideally, all data should be shared so decisions are made based on a complete picture, and the final decision is scientifically sound and based on insightful facts. Insights that are open to interpretation should be flagged. Communication is key when sharing this information. KM processes can assist in ensuring knowledge is shared in the form it is required for the end user and it is communicated, consistent, and findable. This is the real function of the Knowledge Ecosystem.
Future Considerations
Pharma 4.0™
When maximizing the flow of knowledge in an organization, four key factors should be considered to enable a holistic KM program: people, process, content, and technology.
Knowledge is a valuable asset, but often it is not treated that way. Approaches to KM and sometimes data management can vary. This can also result in poor flow of knowledge. Organizations should understand that in the current climate of increasingly complex information generation and large volumes of data, those who manage knowledge well can realize a competitive advantage.
Conclusion
With the use of technology, a huge amount of data and information can be processed. This ability is growing exponentially; however, processing through technology solutions is limited to data and explicit knowledge. Although various technologies have been developed to store, organize, and reuse information, tacit knowledge (the human factor) is still needed to integrate and make sense of this information to create value. Through KM processes (capturing explicit knowledge) and communities of practice connecting people (capturing tacit knowledge), explicit and tacit knowledge become available for use. The more subject matter experts (SMEs) connect across the organization, the more powerful decision-making and the resulting actions will become.
When maximizing the flow of knowledge in an organization, four key factors should be considered to enable a holistic KM program: people, process, content, and technology.
Managing organizational data and knowledge should be a process-driven systematic approach with a life cycle so that data, information, and knowledge are proactively and continuously captured, analyzed, stored, and disseminated. A robust and reliable KM ecosystem integrates product and process information and supports the capture of explicit and tacit knowledge.
As pharmaceutical organizations adopt the Pharma 4.0™ philosophy and embrace the huge amount of data, data connections, structured information, and knowledge in repositories, opportunities for more effective decision-making emerge. This will have a profound effect on how business is managed in the future.