In-Silico Data-Driven Mechanistic Model–Assisted Process Validation

The US Food and Drug Administration (FDA) advocates for the integration of quality by design (QbD) principles throughout the pharmaceutical product development landscape, aiming to elevate both process understanding and product quality. Key challenges to the process control strategy include navigating time- and resource-intensive processes. One solution is digital shadow technology which, when constructed using mechanistic models, offers many benefits throughout the product life cycle.
Successful QbD implementation requires a comprehensive understanding of the intricate relationship between critical quality attributes (CQAs), critical performance indicators (CPIs), key performance indicators (KPIs), and variability in process parameters and raw materials.1
The essential milestones and objectives for achieving QbD include:2, 3
- Defining CQAs, CPIs, and KPIs and their acceptable ranges and identifying process parameters, material attributes, and the ranges that influence them
- Establishing process understanding on how process input parameters impact CQAs, CPIs, and KPIs
- Formulating control strategies and process capability
Challenges to the Process Control Strategy
One of the most formidable challenges on the path to developing the process control strategy lies in the demanding and resource-intensive nature of wet lab experimental process characterization studies (PCS). At the core of this process, the design of experiments (DOE) emerges as a pivotal tool, empowering the development of process knowledge while uncovering the complex multivariate impact of process parameters to product quality and process performance.
Following the design of these experiments, the lab work commences; the scale of the experimentation is typically quite significant, which reflects the complexity of the process tool. For example, a unit operation with five potential process parameters requires around 50 runs to effectively encompass quadratic effects and multivariate interaction effects. Furthermore, even more runs can be required to define the process parameter range used to define the DOE. These runs entail a substantial time investment. For example, each chromatography unit operation can take approximately five to eight hours to finish.
In addition, extensive resources are also required to generate feed materials for the PCS and qualify analytical methods to test PCS samples. This poses a significant challenge and concern to company resources, considering a complete manufacturing process typically includes six to nine unit operations. In some instances, when a different product or process follows similar pathways, a cost-effective “platform” approach is used to streamline process validation and minimize development expenses. When implementing this approach, it is essential to ensure the common elements identified are truly applicable to various products, without compromising the unique characteristics of each product.
Another hurdle encountered by clinical and commercial manufacturing is impact assessments due to process-related deviations. Historically, root cause analysis and process/product assessment heavily relied on the process knowledge of subject matter experts (SMEs), but it takes time for SMEs to acquire the appropriate knowledge. In some cases, the process experience is tacit knowledge that is difficult to transfer to others. This can be detrimental to the manufacturing process because inaccurate conclusions may result in additional deviations reoccurring in subsequent cycles or batches if process history and knowledge are not well maintained.
To target these challenges, this article explores the strategic integration of digital shadow technology into various stages of process validation by defining a digital shadow–assisted process validation framework. A highlight of the article is the demonstration of a powerful surrogate model through the fusion of digital shadow technology and statistical methodologies, which enables more efficient and accurate process characterization.
Digital Strategy (Digital Shadow) with In-Silico Models
As the biopharmaceutical industry embraces Industry 4.0, more options are emerging to address these challenges, with the concept of a digital shadow emerging as a disruptive tool. This concept encompasses a system-level, in-silico model that has proven itself as a predictive powerhouse for evaluating process dynamics and performance. Regulatory authorities have also encouraged the integration of mathematical models to support bioprocess development and manufacturing efforts, leading to a surge of studies centered around in-silico models.4
Statistical and Mechanistic Modeling
Under this concept, two predominant modeling techniques provide the in-silico framework: statistical modeling and mechanistic modeling. Statistical models exhibit exceptional computational efficiency and facilitate automation, thus positioning them as ideal tools for real-time process monitoring and control. However, their predictive scope must be confined to the validated operating space, necessitating a substantial amount of experimental data for model training.5 In contrast, mechanistic models are rooted in physical and biochemical principles. They have been a standard method in chemical engineering for decades and continue to advance within the biopharmaceutical industry.6, 7
Mechanistic models afford profound and scientific process understanding derived from principles governed by natural laws. This imparts longevity to their validity and extends their utility beyond the range of the design space used for model calibration. Consequently, mechanistic models emerge as robust tools for process optimization, deviation analysis, scale-up/-down studies, and process characterization.8 Furthermore, the number of experi-ments required for model calibration and validation is substantially lower than statistical models, showcasing the additional benefit of efficiency and resource economy.
Surrogate Models
In PCS, statistical analysis plays a crucial role in determining how process input parameters impact CQAs, CPIs, and KPIs. A surrogate model is a combination of both modeling methods. In this approach, a mechanistic model is used to assist in conducting DOE studies alongside laboratory experiments. The result is then input into a statistical model for data analysis, leading to the formulation of prediction expressions for CQAs, CPIs, and KPIs. The surrogate model harnesses the advantages of both modeling methods while mitigating their respective limitations.
As the biopharmaceutical industry embraces Industry 4.0, more options are emerging to address these challenges, with the concept of a digital shadow emerging as a disruptive tool. This concept encompasses a system-level, in-silico model that has proven itself as a predictive powerhouse for evaluating process dynamics and performance.
Digital Shadow–Assisted Process Validation
To achieve the milestones in QbD, a robust and systematic process validation framework is imperative and would include:9
- Process design with scaled-down models
- Design production process and process control to ensure that the drug substances/products meet safety, identity, strength, purity, and quality targets
- Build and maintain process knowledge and understanding
- Process qualification with at-scale runs
- Demonstrate the process can consistently produce drug substances/products with target product qualities
- Demonstrate that the commercial manufacturing process can consistently meet the predetermined process performance with the established process control strategy
- Continued process verification during commercial operations
- Continued assurance that the process remains in a state of control (the validated state) during routine commercial manufacturing.
In many of the previously mentioned steps, a digital shadow can be implemented alongside lab work to significantly reduce the resources needed to improve process understanding and establish process control strategy. We propose a digital shadow–assisted process validation framework, which is shown in Figure 1.
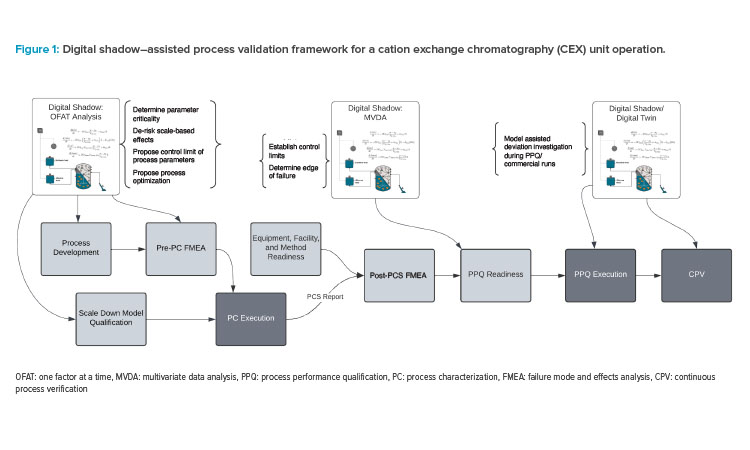
The steps in the process validation workflow where digital shadow assistance can be beneficial are highlighted:
- Process characterization: This step includes process development, scale-down model qualification (SDMQ), and pre-process characterization failure mode effect analysis (FMEA). Digital shadow can be employed for process optimization, determining parameter criticality, defining initial process control strategy, and scale-based effects de-risking.
- Process performance qualification (PPQ) readiness: Digital shadow can assist in establishing control limits and identifying edge-of-failure.
- PPQ execution and continuous process verification: Digital shadow can be used for deviation investigations.
Further details on these functions will be elaborated on in the following sections.
Using Digital Shadow in Process Design
The first stage of process validation is process design, and a successful validation program depends on information and knowledge from process development, clinical, and/or engineering runs.
Typically, the process design phase involves the following key steps:
- Identification of critical aspects: Identify CQAs and CPIs/KPIs and their corresponding specification limits.
- Parameter mapping: Identify the potential critical process parameters, key process parameters, critical material attributes, and key material attributes.
- Model scaling: Design a scale-down model (SDM) and demonstrate that it is representative to the eventual operations at the commercial scale.
- Design of experiments: Formulate a comprehensive DOE and execute it with the qualified SDM.
- Statistical insight: Employ statistical analyses to identify the criticalities and relationship of the process parameters.
- Control strategy formulation: Develop the control strategy based on outcomes of the DOE and process knowledge.
Defining the In-silico Model for a CEX Unit Operation
In the digital shadow–assisted process design, the in-silico model needs to be defined. The steps for defining the model are shown in Figure 2.
- System, column, and biomolecule characterization: Several initial experiments are needed as the first step to gain system-, column-, and biomolecule-level understanding.
- Model selection: The complexity of the model is subsequently determined by specifying the mass transfer assumptions in the mobile phase and stationary phase. This step will determine the calculation time and model accuracy.
- Parameter estimation: Experimental results are used to calibrate/train the model through parameter estimation.
- Model validation: A new set of experiments is used for model validation by running the model with the corresponding process parameters (e.g., load capacity, impurity concentration) and comparing simulation results and experimental data(e.g., yield, purity).
Unit operation characterization
In chromatography, three levels of unit operation characterization need to be done for model calibration and validation:
- System-level characterization: One tracer run is needed for dead volume calculation.
- Column-level characterization: Two tracer runs are needed for the porosity determination and one titration run is needed for ionic capacity.
- Biomolecule-level characterization: In most cases, two elution runs and one high-load run are needed for isotherm parameter calibration.
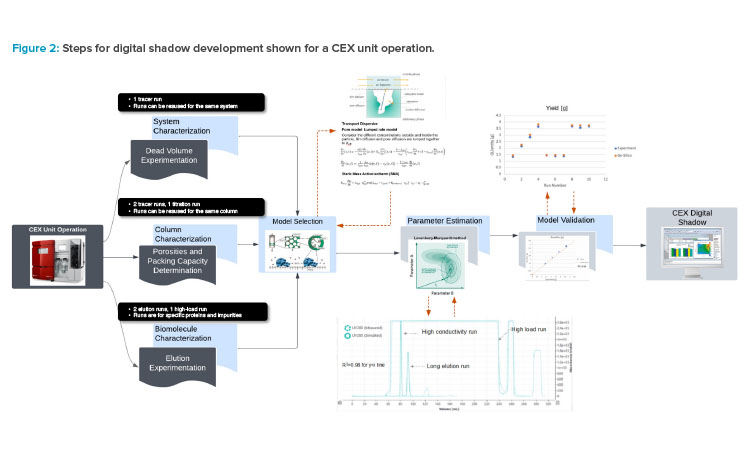
Of all the experiments required, the tracer runs can be performed rapidly and do not need to be repeated for different products, assuming that the same system and columns are used. The elution runs, however, are unique to different products and impurities as they provide information on the physiochemical interactions of the protein and resin.
As the application of mechanistic models is not constrained by the model calibration design space, experiments conducted under any process condition can be used for model validation. For instance, experimental runs conducted during the process development stage can be directly applied, eliminating the need for additional experiments.
Following the validation of the model, it transforms into the digital shadow for this unit operation. A complete digital shadow–assisted process characterization platform is shown in Figure 3.
Digital Shadow–Assisted Process Characterization
After the digital shadow is set up, it can be employed to offer recommendations for the DOE design space with initial runs. Subsequently, an I-optimal DOE is structured to capture both the quadratic and interactive effects of process parameters. The digital shadow is then used to execute the experiments within the DOE.
Building a Surrogate Model
The results from the in-silico runs can be used to build a surrogate model with statistical methods that describe the relationship between the process parameters and, hence, how the process responds to variations in process input parameters. With this in-silico process, the criticality of the process parameters on performance and quality attributes can be evaluated. This approach provides mechanistic understanding for optimizing lab experiment selection, enabling efficient acquisition of valuable results with a minimal number of lab experiments.
The surrogate model can also be used to predict the proven acceptable ranges of the critical process parameters and key process parameters, and then the predicted proven acceptable ranges are confirmed with lab experiments. On top of the impact of single parameters, the surrogate model can also reveal the significance of multivariate interactions. In situations where significant impacts are identified, an edge-of-failure analysis can be carried out. Lab experiments can then be conducted at the identified edge-of-failure parameters to confirm the outcomes from the surrogate model and to make the final refinements to the control strategies. With this approach, the amount of effort, cost, and time needed to develop the process validation control strategy can be reduced by 75% compared to a pure lab-based approach.10
Expected Process Capability Analysis
Upon finalization of the control strategy, the digital shadow can also be used to generate an expected process capability analysis. Employing the Mon-te-Carlo method, a series of random runs with different combinations of process parameter values within the control strategy can be generated and evaluated with the digital shadow. The outcomes from these runs will then be used to conduct a comprehensive process capability assessment.
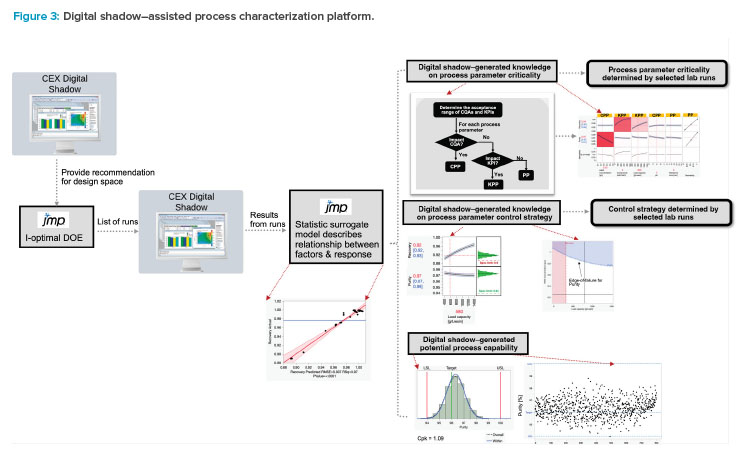
Benefits of Executing PCs With Digital Shadow
Executing PCS with the assistance of digital shadow is expeditious. With the knowledge gained from in-silico runs, the amount of lab work can be significantly reduced, and the in-silico run time is negligible. Typically, a model-assisted DOE can reduce 40%–80% of experiments needed in the upstream domain.11 This provides a significant advantage compared to the conventional practice today that exclusively relies on resource-intensive wet lab experiments for the entire DOE design space.
Oftentimes, PCS works in industry are constrained by limited resources, resulting in a DOE with low statistical power. This, in turn, necessitates more runs during PPQ. However, with the help of digital shadow, the quality of the DOE can be significantly improved with the same resource level, ultimately reducing the number of runs required for PPQ. In cases where a “platform” approach is employed, the digital shadow is also a cost-effective and time-saving tool to identify the commonalities and unique properties of new products.
Digital Shadow in Process Design and Scale-Down Model Qualification
SDMQ is critical for process characterizations. Traditionally, both lab-scale and at-scale runs would be performed and data from both scales is analyzed statistically to assess any scale-induced disparities.
With the digital shadow–assisted SDMQ, a digital model for commercial-scale unit operation needs to be defined. As the process scale changes, there are direct impacts on the fluid dynamic effects caused by differences in equipment geometries. To illustrate in the context of chromatography, columns with identical bed height and smaller inner diameter are normally used as SDMs. In this scenario, column differences (like wall effects, or flow distribution and radial dispersion effect differences) and system differences (like pressure profile or precolumn dispersion and system flow path differences) may lead to different column performance such as peak shape, step yield, and impurity clearance.
It is important to recognize that although fluid dynamic effects are scale dependent, thermodynamic elements (such as protein-resin adsorption iso-therms) remain invariant. As a result, while implementing a mechanistic-based digital shadow, the adsorption model parameters derived from lab-scale calibration experiments can be directly transferred to the commercial scale, requiring only the calibration runs to characterize system and column levels.12, 13
In the case of an observation of discrepancies during chromatography SDMQ, an offset is commonly applied to the SDM. The defined digital shadow with both scales can then assist in the mechanistic understanding of the scaling impact reflected by the offset parameter. One example is from the study by Benner et al. 14 It uses a mechanistic model to elucidate the impact of scale on elution pool volume by systematically analyzing the mass transfer phenomenon under different scales. Leveraging the Peclet number (Pe), the study yielded a fundamental insight of relative significance of axial disper-sion vs. convection across different scales.
Digital Shadow in PPQ and Continued Process Verification
Process-related deviations that occur during PPQ runs and routine commercial manufacturing could impact product quality and/or process performance. For example, in chromatography, factors including column life cycle and process variation can lead to atypical chromatograms, resulting in poor product purity or yield.15 In instances of such deviations, the root cause must be identified swiftly to facilitate corrections in subsequent cycles or batches.
Following the process design, a digital shadow for the commercial unit operation has already been built and validated. Once validated, the digital shadow can be used to support process and/or product impact assessments, which are important elements in process-related deviation investigations. The first step of root cause analysis (RCA) is implementing a tool like a fishbone analysis to identify the potential parameters that might be the underlying cause of the deviation. After the parameters are identified, an inverse modeling method can be employed. This involves systematically altering these identified parameters within the digital shadow to align with the observed unit operation performance thus discerning which factor or factors potentially led to the deviation.16
Furthermore, deviations occurring in a unit operation may stem from variances originating from a preceding unit operation. As a library of digital shadows of distinct unit operations are established for the process, they can be interlinked into an end-to-end, process-level digital shadow. This model can be employed to evaluate how the process parameters of one unit operation influence the outcomes of another. The same inverse modeling method can be used to identify root causes in the connected digital shadow.
RCA is a vital part of continuous process improvement, aimed at identifying and addressing underlying inefficiencies or weaknesses that hinder process optimization and product quality. One of the primary objectives of RCA is the implementation of corrective and preventive actions (CAPAs), which serve to address immediate issues and prevent their recurrence.
Through the application of CAPAs, a more stable and dependable manufacturing process is created. Furthermore, the process understanding gained during RCA, particularly when using the digital shadow for RCA, paved the way for ongoing process refinement. This understanding can be extrapolated not only to rectify the deviating processes but also to enhance other processes, thereby facilitating continuous enhancements.
Conclusion
A digital shadow constructed using mechanistic models offers many benefits and advantages across various stages of the product life cycle. Once the model is established and validated, its utility spans the entirety of the process validation cycle and commercial production, creating a rigorous scientific approach with improved mechanistic understandings. This results in an elevated assurance of product quality while significantly reducing the cost of goods sold (COGS).
Acknowledgements
The authors would like to acknowledge Pia Graf and Nicholas Whitelock from Cytiva; Heath Rushing from Adsurgo, LLC; Thomas Ransohoff from RTI Con-sulting, LLC; Thomas Erdenberger, Huanchun Cui, Joseph Shultz, Christopher VanLang, and Tanveer Ahmed from Resilience; Blair Okita from the University of Virginia; Michael Mylrea from Cyber Team 7; and Behnam Partopour from Sartorius for inspiration and assistance with this article.
*Rui Wheaton and Ahsan Munir contributed equally to this work.