Artificial Intelligence in Drug Target Discovery

The intersection of AI and drug development has ushered in a transformative era, revolutionizing the way researchers approach biomarker/target identification, drug/target interactions, and drug-like molecule design. AI in the life sciences seeks to unravel intricate biological phenomena through systematic assimilation, analysis, and interpretation of expansive and diverse datasets.
The convergence of AI with pharmaceutical sciences holds unprecedented potential from drug target discovery to the safety of drug administration. 2 For example, researchers recently employed graph neural networks to predict antibiotic activity and cytotoxicity for more than 12 million compounds. Using explainable graph algorithms, they then identified substructure-based rationales for compounds with high predicted antibiotic activity and low predicted cytotoxicity. Following empirical testing, they identified a candidate that evades substantial resistance and is selective against methicillin-resistant Staphylococcus aureus (MRSA) and vancomycin-resistant Enterococci (VRE). 3
AI and the Drug Life Cycle
A synergistic relationship between AI and the drug life cycle, including drug target (DT) discovery, will make it possible to streamline therapeutic interventions. It will also pave the way for personalized, patient-centric healthcare approaches. In this article, we delve into the multifaceted potential of AI in revolutionizing one aspect of computer-aided drug design (CADD): AI-enabled DT discovery systems. This includes an outline of the key advancements, challenges, and ethical considerations that underlie this burgeoning frontier. 4
The potential of AI in drug research and development remains substantial, yet its sluggish rate of integration is a cause for concern. This reluctance stems from such factors as entrenched practices and risk aversion within the conservative pharmaceutical industry. However, the hesitancy to fully embrace AI-powered DT development systems may lead to missed opportunities for prospective entity identification, enhanced candidate precision, personalized treatments, and streamlined therapeutic interventions.
As this technology advances, the disparity between the capabilities offered by AI-driven techniques and the status quo may widen, with classical techniques potentially placing patients at a disadvantage by delaying the implementation of more efficient therapy development and delivery strategies.
AI Safety and Efficiency
Recognizing the transformative potential of AI in healthcare, regulators have displayed a proactive stance in ensuring the safety, efficacy, and ethical use of AI-driven technologies in medicine. 5 Thus, it is evident that regulators are playing a constructive role in fostering the integration of AI, and it is other factors within the industry that warrant deeper consideration. Governing and standards bodies are working to ensure the safety, efficacy, and quality of products employing AI within the life cycle framework of ISPE GAMP® 5 Guide: A Risk-Based Approach to Compliant GxP Computerized Systems (Second Edition)6. Examples of this include recent work of the Council of the European Union (EU) 7, 8, 9, International Organization for Standardization/International Electrotechnical Commission (ISO/IEC) 10, and The US Food and Drug Administration’s Center for Drug Evaluation and Research 11, 12, 13call for an urgent modernization of the pharmaceutical industry 14.
AI provides efficiency and entirely new capabilities in analyzing the rapidly increasing type and amount of biological and chemical data employed in identifying potential DTs. It promises to revolutionize the analysis of laboratory data as well as the mining of research publications and digital libraries in the discovery and characterization of biomolecules or pathways associated with disease 15, 16(see Table 1) 17.
Although there are metrics, such as F1 scoring 18, available to report on the efficiency of AI in a particular application, it is hard to overview this in DT discovery. AI-enabled systems and their individual implementations are improving so rapidly that reporting on speed or precision at any one time point is of little value. Also, AI is incredibly powerful in some tasks and very weak in others, so applying AI where it is strong is an important factor in its performance. Nevertheless, the speed and accuracy of AI in facial recognition is a good example of its potential in discovering physicochemical moieties practical in the modulation of a disease phenotype.
Type | Source | Description |
---|---|---|
Life sciences publications | PubMed | More than 36 million citations of biomedical literature from MEDLINE, science journals, and online books |
Publator | Web system providing automatic annotations of biomedical concepts using PubMed abstracts and PMC full-length articles | |
(Small) molecules | ChEMBL | Bioactive drug-like small molecules: containing 2D structures, calculated properties, and abstracted bioactivities |
HMDB | The Human Metabolome Database (HMDB) contains detailed information about small molecule metabolites | |
PubChem | World's largest collection of open chemical information. Search by name, molecular formula, structure, and other | |
Pathways | Reactome | A curated resource of core systems, pathways, and reactions in human biology |
WikiPathways | Provides an open and public collection of pathway maps created and curated by the community in a wiki-like style | |
Ontologies | Mondo | A semi-automatically constructed ontology that merges in multiple disease resources in a harmonized ontology |
Genes | Cellosaurus | A knowledge resource on cell lines. It attempts to describe all cell lines used in biomedical research and production |
ClinVar | An open archive of reports on the relationships between human variations and phenotypes, with supporting evidence | |
Ensembl | Provides a centralized resource for researchers studying human genomes and other vertebrates and model organisms | |
NCBI Gene | Gene-specific information on genomes completely sequenced, in active research, or scheduled for analysis | |
Proteins | IUPHAR Compendium | Details molecular, biophysical, and pharma properties of e.g., mammalian ion and cyclic nucleotide-modulated channels |
String | A comprehensive database from sources of known and predicted, physical and functional protein-protein interplay | |
UniProt | The central hub for the collection of functional information on proteins, with accurate, consistent, and rich annotation | |
Drug development information | Drug Central | Info on active ingredient chemicals, pharmaceutical products, drug mode of action, indications, and pharmacologic action |
Open Targets | Platform that builds and scores target-disease associations and annotations for target identification and prioritization |
AI-driven tools process and analyze highly structured data from multiple sources, such as analytical instrumentation, instrumentation tables, and human-generated data. But what could possibly be the most amazing aspect of AI-driven data analysis, is its ability to process unstructured data, such as raw text from articles and clinical notes, complex spectral data from analytics, and nucleic acid or gene expression sequences and profiles. In DT development, automating data analysis with AI can lead to a level of efficiency that has never before seen in life sciences research.
AI algorithms contribute to the identification and validation of potential drug targets with unprecedented efficiency, beginning with accelerating traditional approaches to DT discovery design. AI improves the wet-lab affinity measurement and comparative profiling approaches employing chemogenomic libraries using high-throughput techniques to rapidly screen thousands of possibilities. However, AI has revolutionized data-mining-driven approaches in 1) multiomic gene-disease association methods by analyzing multiple types of biological data (omics), and 2) computational structure-based approaches examining docking and pharmacophores. These latter approaches rely heavily on diverse biological datasets (see Table 1) 17.
AI’s power expedites the selection of promising therapeutic modes and specific candidates. It also enhances the precision of subsequent drug delivery strategies. Addressing the interconnected nature of therapeutic mode, specific candidate properties, and delivery strategy accelerates the development process, mitigates risks, and advances the paradigm of precision medicine in the pharmaceutical domain.
Therapy Targets: An Evolving Discipline
A therapy target is a molecule or system in the body directly associated with a particular disease that can be modulated by a therapy. Target identification is increasingly important in therapy discovery following recent innovations in assay and experimental technologies. For example, in computational structure-based drug design (SBDD), the constitution of new pharmaceutical agents follows the 3D structures of biological targets. By understanding the structure of a target molecule at the molecular level, ligands are proposed that can interact with the target in a way that may modulate its function.
Some drugs, such as aspirin, operate through an irreversible covalent modification of the target. However, DTs typically involve the therapeutic active ligand and biological target (usually protein) molecular docking. Once bound, small molecule ligands either inhibit the binding of other ligands or allosterically adjust the target’s conformational ensemble.
Investigating these more common, supramolecular (non-ionic/covalent binding) interactions between biological molecules can reveal important bindings, signaling pathways, critical nodes, or chemical reaction catalyzers in disease-related networks. The list of specific systems supporting such potential targets is growing (see Table 2) and all are now integral in the field of DT discovery.
Common pharmacodynamics of the interactions between a drug and its biological target typically involve receptors, enzymes, or ion channels. However, other therapeutic approaches involve the discovery of targets as well. Identification of a defective gene is a form of target discovery prerequisite to gene editing or gene replacement therapies. For example, mutations in the cystic fibrosis transmembrane conductance regulator (CFTR) gene are therapeutic targets in patients with cystic fibrosis 19. The primary therapy approach here is not the use of a drug ligand, but to introduce a functional copy of the CFTR gene into patients’ cells.
System | Description |
---|---|
Protein-protein interaction (PPI) | Small molecules or biologics can be designed to interfere with specific protein-protein interactions to disrupt disease-related pathways. |
Molecule docking | Docking studies use computational techniques to predict how molecules, such as a drug and protein, will interact at the atomic level. |
Chemical reactions' catalyzers | Catalysts, such as enzymes, can be targeted to modulate metabolic pathways. Enzyme inhibitors or activators can regulate these reactions. |
G protein-coupled receptors (GPCRs) | GPCRs are involved in numerous physiological processes. Drugs targeting GPCRs can modulate these processes to therapeutic effect. |
Ion channels | Drugs targeting the proteins in ion channels can regulate the flow of ions across cell membranes, influencing electrical signaling. |
Nucleic acids | Drugs that target DNA or RNA can inhibit replication in pathogens or to modify cellular gene expression in therapeutic applications. |
Receptor tyrosine kinases (RTKs) | RTKs are cell surface receptors that can be targeted by drugs to inhibit or stimulate cell signaling influencing e.g., cell division. |
Transporter proteins | Drugs designed to interact with membrane transporters can modulate the uptake of specific compounds to a therapeutic effect. |
Ligand-gated ion channels | Receptors involved in fast synaptic transmission in the nervous system can be targeted to produce drugs like anaesthetics and anti-epileptics. |
Structural proteins | Genetic disorders can cause abnormalities in structural proteins. Drugs or gene therapies can stabilize or modify them or their function. |
Signalling pathways | Rather than targeting individual proteins, some drugs aim to modulate an entire biochemical cascade of events known as a signalling pathway. |
Epigenetic targets | Epigenetic modifications are genetic alterations regulating gene expression not due to changes in DNA sequence. |
Function | Description |
---|---|
Causality detection | Discovers unknown interactions by identifying dependent and/or independent variables, as well as hidden dependencies. |
Data comparison | Compares simultaneous and parallel data from multiple active moieties or components in both time-series and event data sets. |
Dimensional reduction | Dimensional reduction transforms a number of possibly correlated variables into a smaller number of uncorrelated variables, simplifying complexity while retaining patterns. |
Dimensional analysis | The analysis of the relationships between different objects by identifying their essential features and tracking these dimensions as it places new data in the appropriate dimension. |
Pattern recognition | Identifying specific behaviors or motifs over large amounts of data. By establishing a smaller dataset as a model, it finds similar patterns along the full sequence of information. |
Target prediction | This is the process of calculating the most suitable values for target variables based on inputs from the modeled predictor parameters. |
Clustering and classification | Both supervised and unsupervised algorithms reveal groups in datasets by calculating distances in a multidimensional space. |
The 3D structure of a protein is often required in DT identification. Conventional techniques have resulted in such virtual libraries of the 3D structure of proteins as the open-source Protein Data Bank 20. This comprehensive virtual repository provides 3D structural data of large biological molecules such as proteins and nucleic acids 21. When the target proteins’ 3D structures are unavailable, computational methods such as comparative or homology modeling 22, threading, and ab initio (from first principles) modeling 23, 24have been successful in determining the structures of proteins from their sequences (see Tables 1–3).
Ab initio protein modeling is a computational approach used to predict the three-dimensional structure of a protein based solely on its amino acid sequence and the physical principles governing protein folding. For example, the deep learning–based AlphaFold employs neural networks in a type of machine learning (ML) to perform such modeling, and its source code is freely available 26.
Although by some measurements AlphaFold predictions are remarkably accurate, some note that they do not account for the presence of ambient ligands, ions, covalent modifications, or environmental conditions. In fact, Paul Adams, Associate Laboratory Director for Biosciences at Berkeley Lab, recently remarked, “However, if a researcher wants to study ligand docking for SBDD, there is no substitute for experimental data that provides a higher confidence in amino-acid side chain conformation” 27.
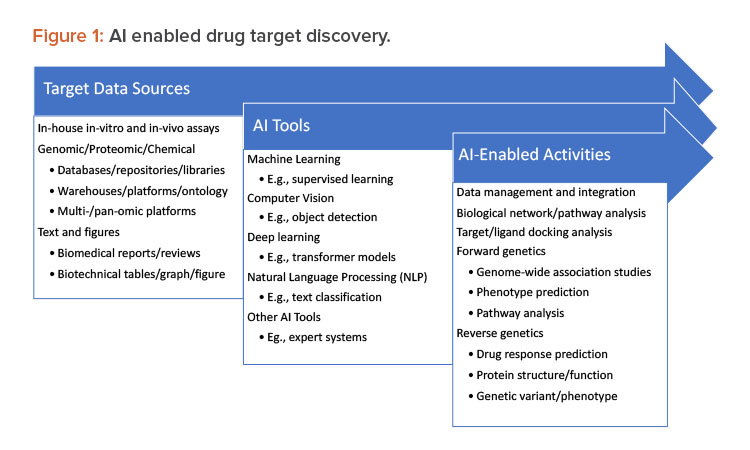
Ligand sampling is a computational method to predict and optimize the binding affinity and specificity between drugs and targets. It explores the conformational space of a ligand from its pharmacophore to identify energetically favorable interactions within the target protein. Many software packages are now available to support either systematic or stochastic ligand sampling approaches.
Unintended pharmacology or “off-target” effects are often caused by a drug interacting with proteins or receptors other than its intended target. These side effects or adverse drug reactions can now be analyzed via AI-enabled meta-analysis of the candidate therapy’s published primary and cross-reactivity. This analysis can be used to map a drug’s overall biological activity and targets—suggesting comprehensive physiological effects and clinical outcomes.
Through exhaustive analysis of both research literature and clinical diagnostic data, AI deepens understandings of demographic and individual patient biology. In personalized, or precision, medicines such analysis can help to identify DTs (this includes beyond universal and subpopulation-specific groups to individual patients). In supporting the repurposing of approved medications for use in other indications, ML algorithms perceive patterns in existing medication-related biological data and link them to even targets of unrelated disease 28, 29.
Progression of Computer-Assisted Target Identification
Software valuable in pharmaceutical target discovery has been developed since the 1970s: Many locally developed open- and closed-source pipelines, libraries, plugins, web resources, methods, algorithms, software, and user interfaces have been created. These include products providing for the analysis, storage, and interpretation of biological data. Support of evolving fields like computational biology is provided by a number of available programs, including software and tools for sequence analysis (BLAST 30), structural biology (PyMOL 31), genome annotation (Artemis 32), next-gen sequencing (HISAT2 33), proteomics (MaxQuant 34), and cheminformatics (RDKit 35).
Software supporting molecular mechanics modeling and computer-assisted organic synthesis are used in organic chemistry to design and predict both small and large molecule reactions. It can include the supramolecular chemistries important in many target bindings 36. In support of them, databases of commercially available starting materials provide practical data for target discovery experimentation 25, 37.
Statistical methods have long been used to identify patterns in data from a DT candidate’s measured genotypic or phenotypic properties as compared to the ideal properties. Results of such searches indicate genes, gene products, or bodily systems as potential therapeutic targets. Examples of early work in this field include genome-wide association studies (GWAS). Their aim is to identify associations of genotypes with phenotypes by testing for differences in the allele frequency of genetic variants between individuals who are related but differ phenotypically 38. A commonly stated goal for GWAS is to use the identified associations to provide a starting point for investigating potential therapeutic interventions.
AI in Target Discovery and Analysis
A tremendous amount and type of data is generated in analyzing the multiple physicochemical and biological systems associated with discovering a candidate biomarker and pursuing its correlation with disease. Similar data aids in the identification of novel therapeutic targets—in particular, disease. This is done by predicting the ligandability of specific regions, as well as in discovering new targets for existing drugs.
In target discovery, analysis, and validation, three distinct and significant steps now appear:
- AI-assisted governance of data from research experiments, libraries, and crowd-sourced databases of compounds, polynucleotides, and proteins
- AI-assisted organization and harmonization of candidate target and ligand structural classification, relationships, and labeling (e.g., 0D–3D)
- Application of AI methods in preclinical studies (see Figure 1).
Target ligand discovery and characterization increasingly involves a combination of experimental and computational approaches. These approaches require interdisciplinary collaboration between chemists, biologists, pharmacologists, and data professionals. Examples include the actionable conclusions now provided by AI in multiomic studies. 39
The number of analytical approaches employing tools like AI-empowered high-throughput screening (HTS) and high-content analysis (HCA) is large and growing. HTS allows researchers to rapidly screen thousands of compounds against a candidate biological target (e.g., a protein or cellular pathway) and reveal which responds to treatment with a particular compound. For example, affinity-based methods can be used to isolate and confirm a candidate target that interacts with the bioactive compounds.
HCA can collect data simultaneously on such multiple cellular parameters as cell morphology, protein expression, and subcellular localization. AI supports analysis of this multiparametric approach to generate phenotypic profiles correlating with disease states or treatment responses. AI-supported HCA enables the analysis of dynamic processes within cells, in a real-time, high-throughput manner, revealing candidate biomarkers or targets actively involved in disease states or drug responses.
HTS provides a broad initial screen to identify targets of interest, whereas HCA provides detailed, contextual information about the activity of a therapy on cellular processes, which helps identify candidate biomarkers and targets 40. For example, Kupczyk et al. used supervised machine and deep learning models to calculate mathematical scores from training databases to create the nodes of the neural networks. The results were then used as a reference to organize the HCA data of the experimental set into groups called classes or classifiers 41.
ML was the first AI tool applied to predicting target–ligand interactions. However, deep neural networks have been shown to outperform both traditional physics-based and knowledge-based ML models. For example, the PotentialNet family of graph convolutions has demonstrated power in predicting the binding affinity of targets/ligands. 42
AI Algorithms
We are seeing such AI algorithms playing a pivotal role in the discovery and analysis of potential DTs. Their proficiency in handling vast and varied datasets, discerning intricate patterns, and generating precise classifications or forecasts revolutionizes traditional, human-dependent approaches 43. This transformation empowers researchers with rapid, robust, and statistically unbiased systems (managed by expert AI methodologies) for identifying and assessing potential targets, ushering in a new era of efficiency and accuracy in drug research. Examples of AI algorithms employed in target discovery and analysis within the pharmaceutical industry include support vector machines (SVMs) 44, random forests 45, and neural networks 46. These demonstrate significant promise in enhancing the selection and evaluation of potential DTs (see Table 3). A summary of algorithms and areas in which they can assist are given next.
Computational Docking and Virtual Screening
AutoDock Suite is a growing collection of computational docking and virtual screening methods used in the exploration of the basic mechanisms of biomolecular structure and function that has proven to be powerful in structure-based drug discovery. Over the years, it has been constantly improved and modified to add new functionalities and features.
The latest version of the AutoDock engine is AutoDock-GPU 47. GPU architectures employing deep learning applications now benefit from new processor types, such as Tensor Core Units. In fact, a method to improve execution time of a core function of AutoDock-GPU by leveraging modern Tensor Core Units has been proposed. 48
In Silico Modeling
Comprehensive in silico techniques using ML and deep learning algorithms model properties, activities, and interactions for potential disease modulating effects. These include bodily components, such as tissues, cell and vesicle surfaces, organelles, blood, and interstitial fluids. The power of this was demonstrated by Insilico Medicine in a phase II clinical trial of its AI-discovered drug, INS018055. 49
Multivariate Analysis
Automated and high-throughput techniques provide measurement of several distinct variables in thousands of unique experimental samples. Variables in biological systems are typically related to various degrees, and so the enormous data sets are multiparametric and sometimes of high dimensionality. However, for historic reasons, they have not always been analyzed as such. Accurate results are ultimately dependent on multivariate analysis (MVA). 43, 50
MVA examines the relationships between multiple variables, which can be challenging using traditional statistical methods. AI-enabled MVA reveals the specific relationships, dependencies, interactions, and feedback within these complex and dynamic biological systems. It provides needed approaches to detect and model numerous interconnected factors to provide an accurate interpretation of complex realities. Supported by power computing and cloud capabilities, AI approaches empowered by MVA provide timely predictions, understandings, outliers, pattern recognition, and recommendations.
Examples of this power in currently available drug discovery tools include an open-access ML program called ConPLex. Developed by researchers at MIT, ConPLex predicts DT interactions. It requires only the sequences of the system’s proteins and simple descriptions of the candidate drugs to provide a sequence-based prediction of DT interactions. 51
Network-Based Sampling
AI-Bind is an AI model that combines network-based sampling with unsupervised pre-training to improve binding predictions for novel proteins and chemical ligands. It is a deep learning DT combination identification algorithm with promise in drug discovery 52. In 2018, Öztürk et al. proposed the first deep learning model for predicting binding affinity between drugs and targets. DeepDTA was a good start, and it has inspired a number of deep learning–based programs, including WideDTA 53and DeepAffinity. 54
Data Resource Searching
Searching compound-target bioactivity data resources can be difficult due to non-standardized and heterogeneous assay types, variability in analytical measurements, and distinct methods for data organization and storage, for molecular structure and motif depiction, and for labels, descriptors, and other terminology. An open-data web platform, DT Commons, features tools to assist in crowd-sourced compound-target bioactivity data annotation, standardization, curation, and intra-resource integration. 55
Recently, powerful commercially available resources have appeared to support the ingestion and integration of varied datasets for use by AI-enabled processing. ONTOFORCE’s DISQOVER is one such platform. It integrates public, private, and licensed datasets to provide a single interface with linked data for optimized data exploration and search. 56
In Silico Screening
In the field of chemoinformatics, neural networks have been used for in silico screening through chemical modeling for years. We are now seeing that, based on further application of AI techniques, it is promising much more powerful analyses. This includes employing virtual libraries representing a much larger chemical space. 57, 58
Property Prediction
ML models can now predict the properties of new compounds in CADD. This is done by employing both measured features of the chemical and purely theoretical descriptors derived from its chemical graph (a graph theoretical representation of a molecule) or 3D structure data 59. A number of powerful tools for exploring libraries for cheminformatics and related applications are becoming available. 60
Examples of AI in Biopharmaceutical Discovery
The activity of immunogens, receptor traps, and enzymes are often mediated by a small number of functional residues, with their domains properly presented by the overall protein structure. We have seen how AI-empowered AlphaFold can predict protein structures from amino acid sequences. This success created hope that such neural networks, trained on well-characterized protein sequences and structures, could also help create novel proteins with understood functionality.
GPT4
Open AI’s GPT4 is a type of generative AI primarily designed for natural language processing. Such applications are now demonstrating capability in the design of therapeutically functional proteins from simple molecular specifications. This innovative approach, known as protein language model transfer, involves adapting the underlying algorithms to interpret and generate protein sequences based on simplified molecular descriptions. The model is trained on datasets containing information about known protein sequences and their associated functions.
BioGPT
The success of GPT4 inspired the development of bio-specific models such as BioGPT, a pre-trained language model that provides text mining and generation for biomedical domain research 61. For example, we are seeing the design of novel protein structures containing prespecified functional sites in an effective orientation customized for a particular use or disease therapy. 62
With this training, BioGPT can generate new protein sequences based on provided molecular specifications. These generated sequences undergo evaluation using computational models and simulations to assess their potential for therapeutically functional properties. Nevertheless, experimental validation in the laboratory remains a critical step in verifying the actual functionality and safety of designed proteins.
It is valuable to realize that AI supports new, previously impossible, capabilities and can radically speed up existing data processing activities and applications.
Chimeric Antigen Receptor T cell Technology
ML techniques enabled researchers at University of California, San Francisco—in collaboration with a team at IBM Research—to expand chimeric antigen receptor T cell (CAR T) technology and make design activities more quantitative and predictive of functionality. First, they constructed a library of CAR Ts containing synthetic costimulatory domains, built from combinations of signaling motifs. Then, using neural networks, they discovered key design rules of the system, which they describe as the ability to “decode the combinatorial grammar of CAR signaling motifs,” 63. This work illustrates how ML tools employing libraries of domains support the engineering of receptors with desired phenotypes. 63
ProSurfScan
ProSurfScan is a new commercially available program to model the compatibility and binding mode of a candidate on different regions of a protein surface 64. There, the protein surface is represented as a graph of nodes defining local supramolecular interaction features. Convolutional neural networks can then agnostically estimate candidate interactions with distinct regions on the protein surface.
Integrated Biosciences
Researchers at the University of California, Santa Barbara are collaborating with Integrated Biosciences, a biotech combining synthetic biology and ML to target aging. Maxwell Wilson, co-founder of Integrated Biosciences and assistant professor at University of California, Santa Barbara. explains, “Our synthetic biology platform enables previously impossible drug discovery because it avoids inducing the collateral damage caused by chemical poisons, enabling us to observe direct effects of drug candidates on the cell’s stress response and perform fast and accurate target deconvolution” 65.
Emerging Therapeutic Modalities and Technologies
We are seeing remarkable developments in advanced therapy medicinal products (ATMPs) therapeutic entities, the means to deliver them, and the technologies employed in their production. AI is becoming essential in handling the amount and type of data coming from the many high-throughput and high-density analytics and screenings employed here 66. Deep learning algorithms such as recurrent neural networks are well-suited for analyzing massive amounts of multivariate time-series data. These algorithms assist in tracking changes over time from assays of cell type-specific responses. This includes the expression of specific genes from cultured cells and biobank submissions.
AI’s deep learning supports a high-level analysis of cell motion, microfluidics, and panomics. New single-cell analysis techniques allow the study of an individual cell’s behavior in tissue and omic contexts. This leads to a deeper understanding of cellular heterogeneity and disease mechanisms. There, AI is providing a deep point-to-point analysis of hundreds of individual cells. Such an extension of single-cell multiomics is yielding more insightful systems understanding than the results of the averages of millions of cells. Recently, improvements in single cell–whole genome sequencing are showing promise in tumors that display a mosaic of genetic variation, or subclonal mutations that make the tumor more aggressive 67. One can only imagine the understanding to be revealed as AI classifiers are applied to this data.
There are many other AI-powered applications aiding in therapeutic candidate and DT understanding, such as virtual reality and augmented reality. These are allowing us to visualize and even interact with complex biological structures and datasets. Such capability was reviewed in a Computer Vision and Pattern Recognition Conference June 2024 in Settle 68.
Finally, it is valuable to realize that AI supports new, previously impossible, capabilities and can radically speed up existing data processing activities and applications. For example, AI-driven natural language processing does not really change the nature of literature review, but it dramatically reduces the time involved in examining and summarizing vast amounts of research and medical literature.
Employing Evidence-Based Associations
By examining the structural, biological, or functional similarity of putative DT in diseases of related ontology, a new initiative, The Disease Ontology, promises an open-source tool for the integration of biomedical data associated with human disease features and mechanisms. It hopes to serve as a reference framework for multiscale biomedical data integration and analysis that will help strengthen the disease information ecosystem 69. In linking disparate datasets through disease concepts, it promises to provide a computable structure of inheritable, environmental, and infectious origins of human disease.
AI can efficiently enable comprehensive multiparametric collection, development, and analysis of the enormous sets of emerging research data with the vast body of biomedical literature, clinical trial data, electronic health records, and other sources. This aids in identifying potential DT in specific patient populations and even personalized medicines. By searching through various real-world patient data, including published off-label use, pointing out the many primary and off-target clinical effects of a drug, AI can help predict candidates in the context of complex endocrine, immune, or metabolic pathways. ML modeling of the multiplex interaction networks of existing therapeutic entity activity with novel candidate DT and disease modulators aids in better understanding the relationships between them.
Challenges
The lack of transparency (“black boxes”) in some AI algorithms makes it more difficult to know whether a system is fair, accurate, and complete. An AI system can also be inappropriate based on flawed data sets or assumptions about its application context, user understandings, or user requirements and procedures. Explainability and transparency in algorithms and their updates is desired to ensure accuracy and compliance in outputs, yet they remain difficult to achieve. Collaboration between domain experts, data scientists, and regulators is crucial to establish and maintain the quality, reliability, safety, and alignment of AI systems throughout the entire product life cycle. 70
Regulatory and Validation Requirements
Regulatory requirements for DT discovery are much less stringent than those for clinical trials or drug approval. They are limited to, for example, International Council for Harmonization (ICH) and good laboratory practice guidelines on data integrity and transparency as well as potential public funding requirements for specific reporting and data sharing mandates.
However, the increasing size and complexity of systems are making it more challenging to identify critical functionalities, define appropriate testing, and establish acceptance criteria that meet sponsor internal guidelines for software reliability, robustness, and performance efficiency. Software-as-a-Service (SaaS), risk-based validation, computer software assurance, and Pharma 4.0™ initiatives determined a significant change in validation demands and solutions. And the unique aspects of AI now further this need for development in validation approaches.
Cybersecurity and Internal AI Processes
The growing concern of cybersecurity refers to technologies and policies to protect data, computer systems, and applications against potential threats such as unauthorized data or systems access and attacks. There are actually two categories of security topics associated with AI.
Secure AI refers to the steps taken to ensure the implemented AI systems are secure from such cyberthreat approaches as brute force, denial of service, and information harvesting. It’s about designing AI systems that are protected from unauthorized access or alteration. Both model integrity and data security are topics of secure AI.
On the other hand, AI cybersecurity refers to AI techniques as a tool to detect, prevent, and respond to cyber threats by enabling improved network security, anti-malware, and fraud detection. There, AI tools are used to identify intrusions by either analyzing communications in real-time to detect and respond to threats, or by analyzing historical data to predict system vulnerabilities and avenues of potential attack. Although it’s true that the internal processes of some, even static, AI algorithms can be hard to interpret, this is not a universal characteristic. An emerging standard in the field of AI called the Open Neural Network Exchange (ONNX) is working to address this concern. ONNX aims to create an open ecosystem where AI models can be developed, trained, and deployed across various frameworks and tools, provided by many organizations promoting transparency and interoperability.
Interoperability
Vertical interoperability in the pharmaceutical sector underscores the critical requirement for a standardized repository of algorithmic tools and foundational structures. These are essential for creating and deploying AI-driven applications and methodologies across a diverse range of proposed concepts and use cases within the pharmaceutical realm.
Horizontal interoperability, on the other hand, centers on the demand for high-quality data sourced from a variety of equipment, hardware, networks, and software utilized throughout a drug’s life cycle. AI-based models are particularly sensitive to insufficient, biased, or incomplete data sets. To mitigate this challenge, it is necessary to establish improved standards for data governance, curation, and exchange. Data integrity in drug manufacturing, as outlined in the ISPE GAMP® 5 (Second Edition) guidelines, emphasizes maintaining the accuracy, completeness, and consistency of data throughout its life cycle, ensuring the reliability and compliance of pharmaceutical processes 71.
Transparency
Applications associated with ONNX enable model portability, allowing the same model to be used in different environments. This enhances transparency and also encourages collaboration and sharing of best practices in AI model development. Therefore, the adoption of standards like ONNX is a significant step toward making AI systems more transparent, accountable, and understandable. Advancements in AI technology, coupled with standardized practices, are steadily reducing the opacity associated with some algorithms 72. Further, the interdisciplinary nature of effective implementation necessitates seamless collaboration between data scientists, domain experts, and regulatory professionals to ensure a harmonious integration of these technologies into drug manufacturing processes.
Drug Development
Lastly, there have been published concerns regarding the use of AI in drug development, including considerations of the failure of some clinical trials following AI-supported candidate identification. Although this is obviously concerning, it should be noted that these events are not outside the current statistics of trial success, and that the particular causes for the trial failure have not yet been established. Furthermore, it is implicit that candidate targets, as well as early “hits” or lead candidates, can be examined and verified using any classical means available.
Conclusion
AI has demonstrated significant power in medical research, diagnosis, and therapy commercialization. It aids in explaining biological phenomena through a structured and comprehensive assimilation, analysis, and interpretation of biological big data. This provides researchers and clinicians extraordinary computational capacity in such comprehensive pursuits as those involving panomics. The value of such DT identification is being driven by innovations in both discovery technologies and in such novel therapeutic modes as precision oncology.
AI is empowering the methodologies, applications, and emergent frontiers that underlie drug discovery and delivery throughout the entire drug life cycle 73. The accelerated application of AI in DT identification profoundly enhances our understanding of effective therapeutic interactions, and it expedites the entire process from target discovery to drug manufacturing 74. This accelerated AI-driven approach holds immense value for patients by streamlining the development of more targeted therapies. Ultimately, this will lead to more efficient and personalized treatments with the potential to reduce off-target effects and improve clinical outcomes.