Driving Biopharma Solutions with Digital Technologies

Developing comprehensive digital solutions is crucial for the entire value creation process for pharmaceuticals. A holistic view of the interrelations of product, production process, and plant is becoming increasingly significant. In this context, the application of model-based technologies provides support in drug development, process scale-up, and manufacturing. Furthermore, it accelerates time to market. The prerequisites: adequate software solutions and the willingness to break down silos and bridge gaps between disciplines.
Several often interrelated trends are driving change in the pharmaceutical industry and not only because of the COVID-19 pandemic: achieving quicker production of new drugs, accelerating time to market, and delivering affordable patient treatments. These trends make unprecedented demands in terms of agility, flexibility, and adaptability and must be addressed by science and technology—and this is where digitalization can contribute significantly.
By using digitalization strategies—which contain innovative approaches to discover, develop, and manufacture new drugs—agility is increased and overall development and production time is reduced. The full potential of available technologies, such as simulation or data analytics, could be even better realized by uniting them in a smart way. This would require combining and using data that results from all aspects and phases of the life cycle of a drug, from its development, clinical studies, and production process all the way up through to the responses of patients to their treatments (e.g., desirable side effects).
Digital Twins
A key aspect of the large change process based on digital technologies, also known as digital transformation, is the digital twin. The digital twin is the most exact virtual representation possible of a real system, with all its components, their properties, and functionalities. In the pharmaceutical industry, everything starts with the patient’s needs and the goal is to develop a drug targeting those needs. A process capable of delivering a stable product and engineering a plant that is fit for the process must be established. This is why we speak of several twins—the digital twin of the patient, product, production process, and production plant.
A key challenge for companies active in drug development is to shorten the lead time from early-stage development in the clinical phase to the commercial production scale. Smartly applied digitization is crucial for the entire value creation process. It is no longer enough to just optimize individual steps in the value chain: a holistic approach is required.
The 4 Ps of the Value Chain
The pharmaceutical value chain is based on four fundamental and strongly interconnected elements: the patient, an effective product, an approved production process for that product, and a functioning plant to make that product, all of which work according to the regulations of the authorities. Each of these “4 Ps” can find its virtual representation in a digital twin (see Figure 1).
The trend toward personalized medicine makes patient data increasingly important. The patient’s digital twin would include their genetic properties and personal medical characteristics like metabolic fluxes or drug reactions. The digital twin of a product tailored to the patient’s needs contains information about its molecular structure and properties, its critical quality attributes (CQAs), and its design. The production process—with its individual steps, required equipment, critical process parameters (CPPs), and control and simulation systems—can also be depicted by its own digital twin. The virtual representation of the production plant on which this process is run is based on data regarding building layout; the respective equipment, utilities, modular structures and their properties; piping; and technical building equipment.
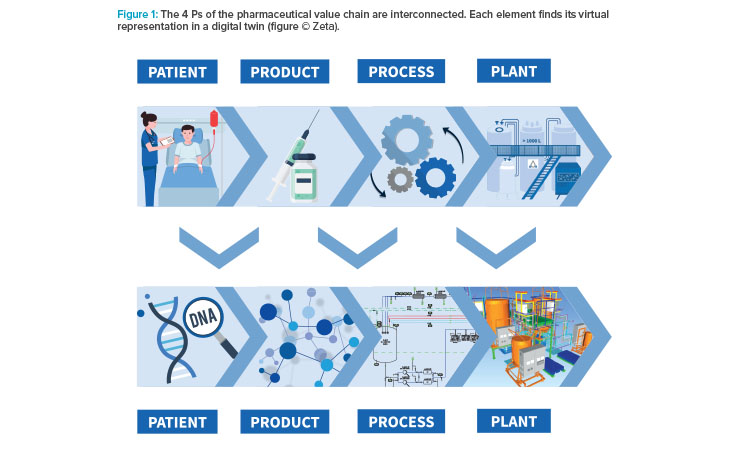
Integrated Engineering for Process and Plant
In the pharmaceutical industry, digital platforms allow for aggregation of massive amounts of data from a variety of sources. During production, for example, data on the current state of the plant and product are generated by metrological instrumentation and the records required by GMP are made digitally in the electronic batch record to safeguard the product’s quality. Data from operations alone are often not sufficient for an efficient and reasonably usable digital twin of a system for maintenance, energy, or production optimization. Data that have already been generated in the course of system planning (e.g., 3D data, extensive information from piping and instrumentation diagrams [P&IDs], component specifications, and electrical planning data), so-called metadata, serve as a valuable addition here.
The integration of data derived from process monitoring and engineering results in a digital twin that is useful for a number of applications. The pre-requisite for this integration of the virtual, digital world into the real, physical world is uniform data from all engineering disciplines through all project phases (concept, basic, and detail engineering).
However, the creation of harmonized data—the digital twin—is impossible as long as the data only exist in silos, as is still very often the case. During investment projects to create a manufacturing facility for a new drug, a number of project partners are included and many aspects have to be covered—engineering, process scaling, applying the needs of cGMP—and multiple disciplines have to be integrated, such as process engineering, 3D design, electrical engineering, automation, and qualification. Although state-of-the-art technology may be used (e.g., computer-aided design software for creating P&IDs, 3D models, or electrical wiring diagrams), the respective tools are usually operated separately by the project partners and do not blend data with each other. By using digital, integrated engineering, such data silos are avoided and uniform data are generated. Specific product life-cycle management solutions cover the workflows from concept design of a plant to basic and detail engineering (P&ID, electrical engineering, 3D design, electronic qualification and allow all project partners to deliver harmonized data from all disciplines. These tools serve as one common software landscape for all project partners and enable data input, data management, and data use. All engineering workflows and user-oriented front ends are covered.
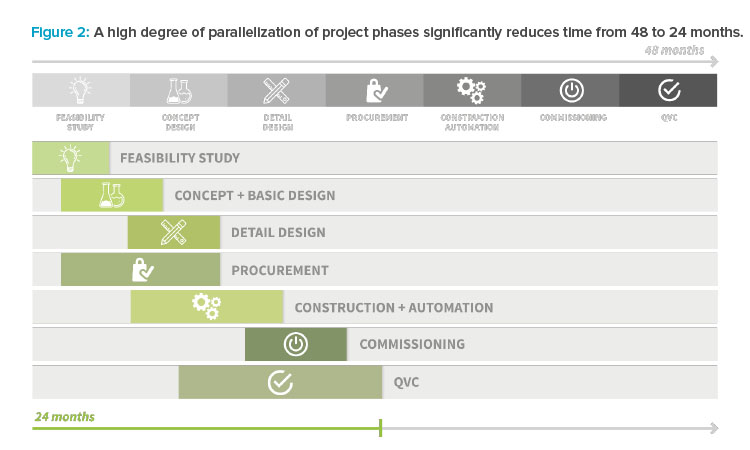
Combining data input of all project partners in real time delivers a harmonized data set: the digital twin of the process and the plant. This harmonization of data significantly reduces project risks due to 100% transparency for all project partners at all times. This transparency results in benefits in many areas, such as change management. Unavoidable changes of equipment at later stage of engineering require massive effort, as they affect all disciplines: Changing a pump in its dimension causes impact, from the 3D design all the way to electrical and automation engineering and qualification work-flows. Communication of the changes to all disciplines are time-consuming and error-prone. Integrated software landscapes allow better management of these changes, because consequences of changes become transparent before the change is approved, and all project partners can follow up on the change in real time in their own domain.
A refinement of the digital twin of the process by including a process simulation offers further advantages in qualification and validation. An example: the possibility to revert to a simulation of plant and process during automation software commissioning allows testing of functionalities (like recipes, control strategies, and interlocks) within a short timeframe and independent of equipment. This results in shorter commissioning times during factory acceptance and site acceptance tests (FATs and SATs). Furthermore, this simulation can serve as the basis for operator training simulations.
Finally, this harmonized data are the basis for future applications in the field of augmented or virtual reality, as it contains all the information about the 3D dimensions, the equipment (tag numbers, spare parts), and its location on the manufacturing site.
Recently, the approach to apply one centralized digital toolchain delivered remarkable results at a project in Vienna, Austria. The project covered the engineering and construction of a downstream processing facility for vaccine production, in accordance with already existing facilities. The scope included civil construction, electrics, HVAC and cleanroom, process equipment, utilities, automation, qualification, and commissioning. To ensure market supply for the human papilloma virus vaccine, the targeted project lead time was 24 months from feasibility study to first production run. To meet the ambitious timeline, the engineering phases were parallelized (see Figure 2).
To handle the complexity increase that resulted from concurrent work, a centralized digital platform was applied. This platform allowed efficient management of the different disciplines and project partners during all project phases. Collaboration between project partners and the end customer was supported, and efficient reviewing of P&IDs and 3D design, including real-time access to the 3D model, was ensured via a collaboration platform. As the project progressed, all documents, specifications, 3D models, wiring diagrams, and automation applications contributed to the digital twin of process and plant. At the end of the project, a comprehensive digital twin of process and plant was available and was used for commissioning tasks as well as for later operations.
This digital twin served as a tool for communication between the project partners and the end customer, and it helped ensure plant usability, a maintenance-friendly design, and appropriate ergonomics. All equipment (including actuators, sensors, and vessels) and their locations were digitally specified and managed, and a complete list of spare parts was available. This data was combined in a mobile application running on smart devices for supporting the maintenance tasks (see Figure 3).
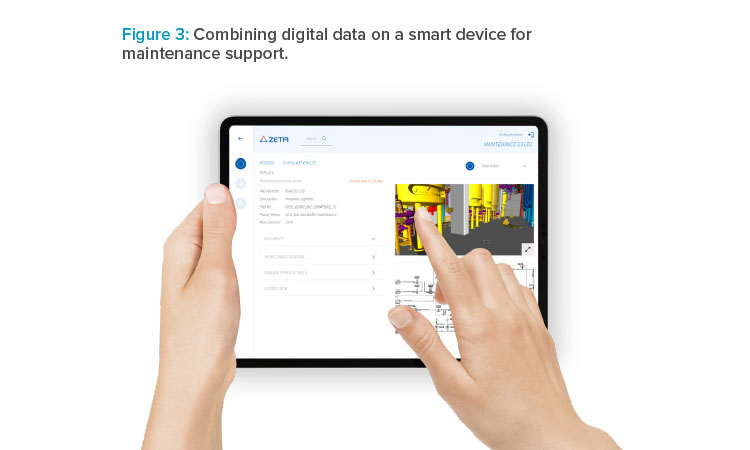
Process Development and Scale-up
Data are also digitally recorded and documented in product and process development. Modeling and computer simulation techniques are increasingly being used. The process, which was initially developed on laboratory scale, is mapped digitally and the result is used for technology transfer. For clinical development, a scale-up is necessary, as larger quantities of product are required, which must be manufactured meeting GMP criteria.
During product and process development, when process conditions are specified on a scientific basis using quality by design (QbD) principles, valuable information is generated and essential criteria are worked out. The CPPs are defined, which has a decisive influence on the CQAs of the product. This implies it is reasonable to develop and optimize the product or process and at the same time derive valuable information for engineering the scale-up (pilot scale) and the manufacturing plant (production scale). Following the approach of FDA industry guidance,1 this data should be used beyond the development stage, which is still not widely applied.
Fermentation in a bioreactor is an example: design parameters have a decisive influence on product quality. On a one-liter scale, a large amount of data that affects the interaction between the product and the process is determined in the laboratory: gassing rates, temperature, pH value, and occur-ring pH jumps. These parameters (design of experiment [DoE]) span a space in which many variations are possible and optimum conditions are evaluated (bioprocess modeling).2 In the next step, a set of parameters is assigned to the design of the production plant. A determined oxygen input, for example, can be achieved in different ways, such as by adjusting the shape and geometry of the agitator or by the design of the gassing device.3
Model-based technologies, as part of the digital process twin, are the key to simulations and many optimization measures. Experimental time is reduced by model-based DoEs during process development and characterization. A further benefit of the models is the possibility of using them in the context of closed-loop process control,4 operator training, or for virtual sensing techniques (soft sensors) that are used to provide alternatives to costly or impractical physical measurement instruments.
Models for Closed-Loop Process Control
The bioprocess model can be described as the digital twin that results from combining data on the production process and the product itself. On account of the QbD approach, DoE-based bioprocess models have been applied in process development for over a decade. In the manufacturing stage, however, process models are not yet applied, even though the exploit of the power of mathematical models was recommended by the FDA in the PAT guideline.1 Following the PAT guideline, future submissions may include new control strategies, such as model predictive control (MPC). MPC resorts to the bioprocess model based on the QbD/DoE approach. To provide a proof of concept, the applicability of DoE-based mathematical models for closed-loop control of a manufacturing process was explored and demonstrated. Using this approach completes a major step in closing the gap between process development and manufacturing.
Closed-Loop Process Control
In the proof of concept previously outlined, a closed-loop control strategy, as depicted in Figure 4, was elaborated. In closed-loop control systems, also known as feedback control systems, process variables are automatically regulated to a desired state. Such systems possess the ability to self-correct without human intervention. Closed-loop control in manufacturing processes is supposed to become even more relevant when moving toward continuous processes.
Establishing Closed-Loop Control Technology
To establish closed-loop process control, a number of software tools are applied. Several workflow steps have to be taken until the application can start:
Modeling/DoE
An applicable process control strategy has to cover the interdependencies of the CPPs and the resulting CQAs. In the experimental setup for a fed-batch cultivation of E. coli in a 60 L GMP-compliant bioreactor equipped with an industrial automation system (DCS), temperature, feeding rate (growth rate), and induction strength were assigned CPPs, and the CQAs were biomass and soluble product titer. For modeling of these interdependencies, a novel software tool was used. This tool combines a parametric model, covering the basic principles within the process (mass and energy balance, for example) with a nonparametric model (machine learning algorithm, artificial neuronal network).2, 5, 7
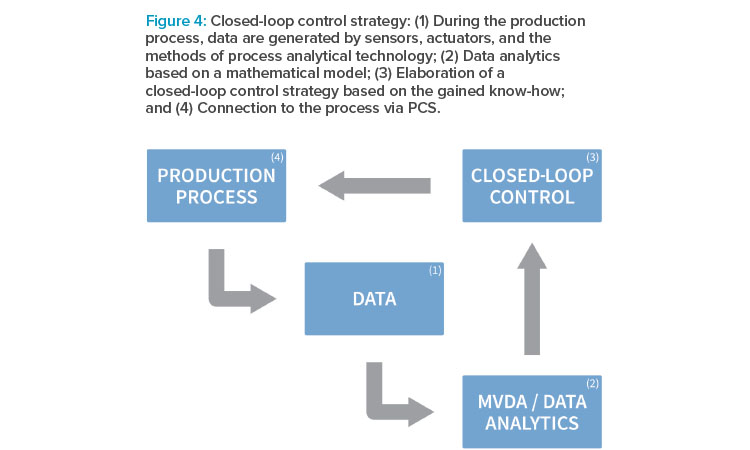
MPC
The MPC is a software that further uses the established model and combines it with an objective function. In principle, it is like a GPS navigation system in a car: the model is the map, the MPC is the device, and the objective function allows for different strategies to follow—shortest route, fastest route, cheapest route. After defining objectives and constraints, the setup is used for optimization. The optimization algorithm calculates the optimal values for the manipulated variables (in this case, temperature, feeding rate, inducer). The software receives the references for these manipulated variables, as well as the current values for the controlled variables (product titer, biomass) from the process control system (PCS). It further calculates the next values for the manipulated variables and transmits them for execution to the PCS.
MPC approach benefits
With the scientific-based approach using intensified DoE, the process is explored in an advanced way. A robust model of superior performance in terms of robustness, accuracy, and reproducibility is generated. The reuse of this elaborate model for closed-loop process control enables the process to run in perfect conditions regarding titer and quality of product.
Starting the building of the model early in process development phase enables model transfer along scale. With minor adaptations, models can be used from small scale up to large scale. Hence, the implementation of MPC ideally starts in development phase when process variations for model calibration are available. This reduces risk, improves plant performance, and assures the intended quality. Furthermore, flexible operation strategies are supported, as changing of boundary conditions while safeguarding the quality of the product is facilitated.
From a cost perspective, it can be concluded that applying MPC at production facilities will significantly improve plant efficiency by improving yield/time and yield/space ratios. It can counteract out of specification (OOS) production and potentially save full production batches.
With the scientific-based approach using intensified DoE, the process is explored in an advanced way.
Conclusion
It is important to overcome the siloed thinking that exists among lab, pilot, and industrial scales. If the appropriate digital twin of the biological process (bioprocess model) has been developed at the small scale, it can be accessed in the planning for the next larger step and has the potential to greatly increase the accuracy of the CPPs at larger scales. Simulated test runs can be performed and fewer test runs at production scale are necessary, which can significantly reduce time to market. Furthermore, the bioprocess digital twin can be used to address a variety of questions about the influence of process parameters, equipment, conditions, safety, and competitiveness.
Plant constructors focus on the technical process and the plant itself. In product development, the focus lies on the bioprocess model combining the product and the process. To get a comprehensive picture, it is necessary to go one step further and combine these approaches. Blending the digital twins of the bioprocess and technical processes together in one simulation environment or platform results in an improved understanding of the interactions between process, product, and plant. It permits the acquisition of information, for example, on energy and mass balances, vessel sizes, and buffer quantities, even before the plant is constructed. This software platform supports the engineering and manufacturing procedures to provide a commercially attractive production process at final scale.
Using a comprehensive picture of the interdependencies of plant, process, and product and an early understanding of their interactions, improved engineering results and a whole range of further advantages are obtained. Model-based technologies allow the sharing of knowledge needed for scale-up, support the implementation of the QbD approach, and allow the application of advanced process control techniques. A significant reduction in time for process development is the result.
Individual digital twins, or process models for single-unit operations, are incorporated into a comprehensive digital twin, a fully integrated process model. An adequate software environment with the respective IT platforms is required for this approach. A comprehensive toolchain, in terms of systems, software, and methodological support in combination with sound engineering expertise, is essential. The challenge lies in creating an overarching concept and architecture. A climate of co-creation between stakeholders, suppliers, partners, and experts is the prerequisite to break up silos and to support fast-track biopharmaceutical drug development and manufacturing. Not least because of the COVID-19 crisis, we have learned how essential the bundling of competencies is to be able to quickly respond to patients’ needs.