Machine Learning Risk and Control Framework

Stakeholders across industries are becoming accustomed to using information technology (IT) systems, applications, and business solutions that feature artificial intelligence (AI) and machine learning (ML). Even though some of these uses show phenomenal performance, thorough risk management is required to ensure quality and regulatory compliance are met within the life sciences industry. By leveraging specialized frameworks and methods, we compiled a holistic framework to dynamically identify, assess, and mitigate risks when AI and ML features are in use.
A Risk-Based Approach to AI and ML
After years in which AI initiatives commonly failed to pass the pilot stage, the operational use of AI applications within the life sciences industry is evolving and rapidly gaining momentum. With a substantial increase in regulatory approvals and AI applications like ChatGPT being commoditized and granted higher levels of autonomy, it is imperative for the life sciences industry to implement a framework to review the risks of and controls for AI to maintain product quality, patient safety, and data integrity.
This article adopts the ICH Q9(R1) risk management process as a basis to address the specific challenges for AI systems. Along this harmonized risk management process, our framework—the ML risk and control framework—builds on recently developed AI methods and concepts to identify and assess the entire risk inventory for a given use case along the life cycle, represented in a risk analysis and mitigation matrix. The resulting framework offers a straightforward structure to continuously manage the complexity of ML-related risks throughout the system life cycle, from concept to operation. Furthermore, due to its ease of use, science-based approach, and transparency, the true value of the ML risk and control framework unfolds during periodic risk review by facilitating understanding and informed decision-making.
Rapidly Evolving AI and the Necessary Risk Management
The field of AI has rapidly evolved. The remarkable and swift transformation has marked an extraordinary pace of progress, driving disruption and fostering a wave of innovation across industries. According to the Stanford Institute for Human-Centered Artificial Intelligence’s annual AI Index Report for 2023, the total number of AI publications has more than doubled since 2010, growing from 200,000 in 2010 to almost 500,000 in 2021.1 The use of AI within the life sciences has gained traction and is accelerating. According to the FDA, the number of approved AI-/ML-enabled medical device approvals increased by a staggering 1,800% since 2015, growing from 29 in 2015 to 521 as of October 2022.2
With the introduction of ChatGPT and other generative AI applications raising public awareness of its power, AI’s potential use cases have also exponentially grown, which brings associated risks.3 Such awareness sparked a surge in desire to exploit the potential of data and data-driven insights backed by numerous pilots and first productive applications.
The urge to integrate AI- and ML-featured technology into the production software system landscape is mirrored by several regulatory initiatives, including FDA discussion papers and European Medicines Agency (EMA) papers: Artificial Intelligence in Drug Manufacturing4 and Using Artificial Intelligence & Machine Learning in the Development of Drug & Biological Products5 from the FDA, and “The Use of Artificial Intelligence (AI) in the Medicinal Product Lifecycle”6 and “Concept Paper on the Revision of Annex 11 of the Guidelines on Good Manufacturing Practice for Medicinal Products – Computerised Systems”7 from the EMA.
However, both current and future initiatives will take time to develop because neither AI nor ML are mentioned in current GMP guidelines and regulations. This is unlike other areas like Medical Devices where some consensus on good ML practices has been established.8 . Conversely, multiple industry guidance documents exist, including the ISPE GAMP® RDI Good Practice Guide: Data Integrity by Design9 and the ISPE GAMP® 5 Guide: A Risk-Based Approach to Compliant GxP Computerized Systems (Second Edition),10 elaborating on the principles for management of AI and ML components and subsystems throughout the life cycle from a high-level perspective.
Although risk management and criticality of operational monitoring are put into focus in these guidance documents, we identified a gap in its practical application. How can an organization manage risks and controls during the concept, project, and operational life cycle phases when there are so many choices in autonomy and control AI- and ML-featured IT systems, applications, and business solutions? As such, we compiled a holistic framework to operationalize the increased risk awareness of AI as exemplified by applications such as large language models or concepts like explainable AI.11 Thus, from our point of view, it is important to establish an industry understanding on:
- The criticality of risks in various contexts of use during initiation of the quality risk management process
- A typical risk inventory along the life cycle of an ML subsystem and its integration into the computerized system landscape
- Suitable and appropriate risk mitigation strategies
- A dynamic process governing the life cycle, ensuring a continued state of control
Foundations and Principles
The International Council for Harmonisation of Technical Requirements for Pharmaceuticals for Human Use (ICH) published its first revision of the ICH Q9 guideline on quality risk management in early 2023 [12]. This guideline “offer[s] a systematic approach to quality risk management [and] serves as a foundation or resource document that is independent of, yet supports, other ICH quality documents and complements existing quality practices, requirements, standards, and guidelines within the pharmaceutical industry and regulatory environment.”12 As such, ICH Q9(R1) provides a high-level process, which is summarized in Figure 1.
The ICH Q9(R1) guidance is applicable to any process and system, agnostic of whether this system comprises ML subsystems. Therefore, from an operational risk management perspective, many questions remain unanswered when considering such components: the impacts and potential risks associated with data, the choice of models and training algorithms for risk management during productive operation, and the control of phenomena such as drift.
- 1The Stanford Institute for Human-Centered Artificial Intelligence. Artificial Intelligence Index Report 2023. Stanford, CA: Stanford University, 2023.
- 2US Food and Drug Administration. “Artificial Intelligence and Machine Learning (AI/ML)-Enabled Medical Devices.” October 2022. www.fda.gov/medical-devices/software-medical-devicesamd/ artificial-intelligence-and-machine-learning-aiml-enabled-medical-devices
- 3Heitmann, M., S. Münch, B. M. Stockton, and F. Blumenthal. “ChatGPT, BARD, and Other Large Language Models Meet Regulated Pharma.” Pharmaceutical Engineering July/August 2023. https://ispe.org/pharmaceutical-engineering/july-august-2023/chatgpt-bard-andother-large-language-models-meet
- 4US Food and Drug Administration. Artificial Intelligence in Drug Manufacturing. Silver Spring, MD: US Food and Drug Administration, 2023. www.fda.gov/media/165743/download
- 5US Food and Drug Administration. Using Artificial Intelligence & Machine Learning in the Development of Drug & Biological Products. Silver Spring, MD: US Food and Drug Administration, 2023. www.fda.gov/media/167973/download?attachment
- 6European Medicines Agency. Committee for Medicinal Products for Human Use. “The Use of Artificial Intelligence (AI) in the Medicinal Product Lifecycle.” July 2023. www.ema.europa.eu/en/use-artificial-intelligence-ai-medicinal-product-lifecycle#current-version-section
- 7European Medicines Agency. GMP/GDP Inspectors Working Group. “Concept Paper on the Revision of Annex 11 of the Guidelines on Good Manufacturing Practice for Medicinal Products – Computerised Systems.” September 2022. www.ema.europa.eu/en/documents/regulatoryprocedural- guideline/concept-paper-revision-annex-11-guidelines-good-manufacturingpractice- medicinal-products_en.pdf
- 8US Food and Drug Administration. “Good Machine Learning Practice for Medical Device Development: Guiding Principles.” October 2021. www.fda.gov/media/153486/download
- 9International Society for Pharmaceutical Engineering. ISPE GAMP® RDI Good Practice Guide: Data Integrity by Design. North Bethesda, MD: International Society for Pharmaceutical Engineering, 2020.
- 10International Society for Pharmaceutical Engineering. ISPE GAMP® 5 Guide: A Risk-Based Approach to Compliant GxP Computerized Systems (Second Edition). North Bethesda, MD: International Society for Pharmaceutical Engineering, 2022.
- 11Altrabsheh, E., M. Heitmann, P. Steinmüller, and B. P. Vinco. “The Road to Explainable AI in GXP-Regulated Areas.” Pharmaceutical Engineering January/February 2023. https://ispe.org/ pharmaceutical-engineering/january-february-2023/road-explainable-ai-gxp-regulated-areas
- 12International Council for Harmonisation of Technical Requirements for Pharmaceuticals for Human Use. “ICH Harmonised Guideline Q9(R1): Quality Risk Management.” Adopted January 2023. https://database.ich.org/sites/default/fi les/ICH_Q9%28R1%29_Guideline_Step4_2022_1219.pdf
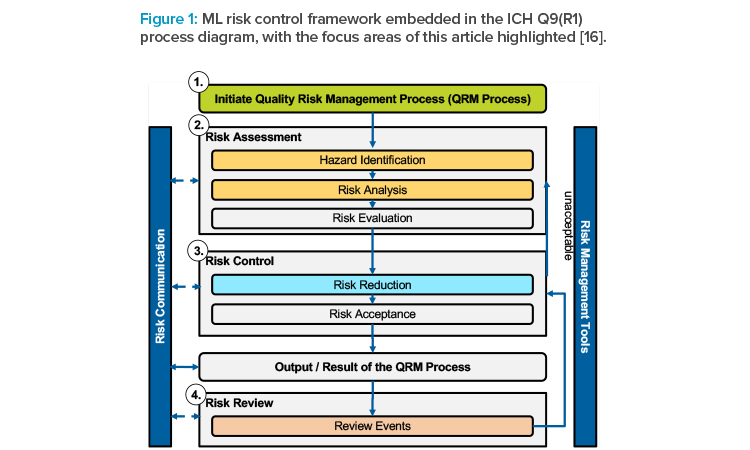
However, with ICH Q9 being the internationally harmonized guideline, we base our ML risk and control framework on key process steps to facilitate compliance with regulatory guidance and thus avoid additional complexity. We considered integrating the Second Draft of the ML Risk Management Framework published by NIST;13 however, because guidance provided in this draft is less specific to the industry than ICH Q9(R1), we focused on the latter.
Because GAMP 5® Second Edition is widely adopted in the industry, we also base our framework on concepts provided in this guideline, particularly those in Appendix D11—Artificial Intelligence and Machine Learning. 10 . Here, GAMP 5® Second Edition suggests a software development life cycle model for the development and computerized system integration of ML subsystems.
The GAMP Machine Learning Sub-System Life Cycle Model comprises three primary phases: concept, project, and operations. We use this model for navigating the risk landscape to facilitate a structured identification of hazards, considering organization-, data-, process-, and methodology-related facets in the area of ML methodology to build a comprehensive risk inventory.
Further guidance has been developed, providing details of organizational aspects and blueprints for decision-making. Specifically, we extend the “AI Maturity Model for GxP Application: A Foundation for AI Validation”14 and include concepts and ideas of the AI governance framework,
- 13National Institute of Standards and Technology. US Department of Commerce. “Artificial Intelligence Risk Management Framework (AI RMF 1.0).” January 2023. doi:10.6028/NIST.AI.100-1
- 10
- 14Erdmann, N., R. Blumenthal, I. Baumann, and M. Kaufmann. “AI Maturity Model for GxP Application: A Foundation for AI Validation.” Pharmaceutical Engineering March/April 2022. https://ispe.org/pharmaceutical-engineering/march-april-2022/ai-maturity-model-gxpapplication-foundation-ai
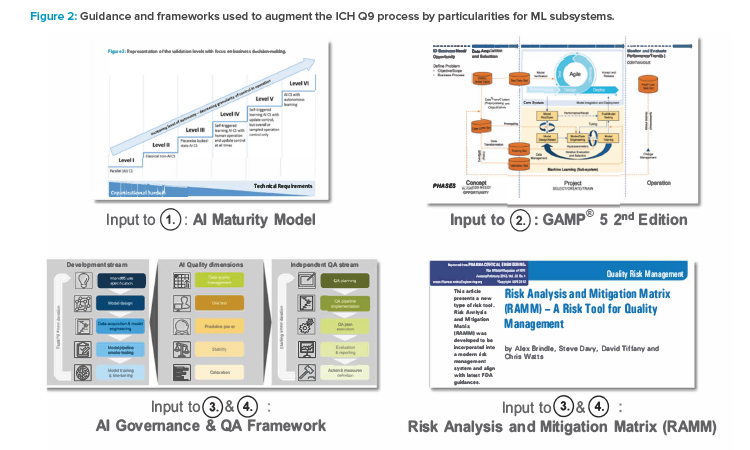
Based on these premises, this article proposes guidance that can be easily followed for the practical implementation of an appropriate ML risk and control framework. It is based on the following general principles, aligned with previously mentioned references and previous work:
- Commensurate effort: The risk and control framework yields solid reasoning on the risk strategy, respecting the organization’s risk tolerance and the risk inherent in the process that is using ML methodology.
- Holistic view: The proposed framework helps identify risks that arise from development and operation of AI/ML models embedded in a computerized system. It integrates accepted data science methodology with the concepts of product quality and patient safety in the regulated areas of the pharmaceutical industry.
- Compatibility with accepted methodology: Risk management is not new—it has been practiced for decades, with a primary focus on classical, non-ML-enabled computerized systems. We aim for compatibility of widely adopted approaches, hence augmenting current approaches to risk control of GxP relevant processes with particularities of ML methodology.
- Dynamic process understanding: Further demonstrating that risk management is iterative, risk assessment will change as more process understanding is gained. This is particularly true for forward-looking methodology. Hence, the effectiveness and adequateness of risk mitigation measures should be assessed regularly to ensure the process is in human control and to unlock further opportunities in the scope and autonomy of the ML solution.
These concepts will be explored further in this article, providing generally applicable, though operational, guidance along key steps in the ICH Q9(R1) risk management process. Similar to the GAMP ML Sub-System Model, this framework is intended to be embedded into an existing risk management process for computerized systems to ensure additional ML-related risks are managed throughout the life cycle of the computerized system.
As with the overall ICH Q9(R1) risk management process, the framework is designed as a structured process to break down the ML-specific challenge of risk management. It is not meant as a one-time exercise and should accompany the model along its life cycle. The framework adds to the following segments to the ICH Q9(R1) process:
- Initiate quality risk management process
- Risk assessment (in particular, hazard identification and risk analysis)
- Risk control (in particular, risk reduction)
- Risk review
These additions are described in detail in the following sections and shown in Figure 2.12 The framework can further be used for extending the set of risk management tools to facilitate effective risk communication.
To illustrate its practical application, we accompany these concepts with the following example of downstream process optimization in biotech active pharmaceutical ingredients (APIs) through the quality control of a chromatography step. The objective of this ML application is to determine the optimal point to start and end the capture of the APIs, splitting undesired impurities from the downstream manufacturing (see Figure 3). The operator uses this information to execute the API capturing. The ML model is trained on historic data, balancing quality objectives on the API’s purity and optimized yield of the resulting batch.13
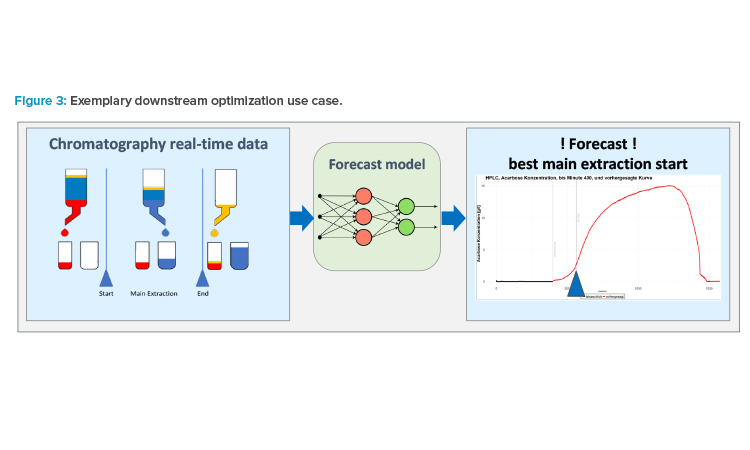
Initiate Quality Risk Management Process
In alignment with ICH Q9(R1), our risk management framework starts with the initiation of the quality risk management process. For ML applications, it is recommended to perform this during the initial planning phase by leveraging the AI Maturity Model.14 The AI Maturity Model can be used to define expected autonomy as well as the control design level that together form the target operating model in the first productive ML-featured layout (minimal viable product, or MVP). Thereby, the autonomy describes the “feasibility to automatically perform updates and facilitate improvements.” The control design is the “capability of the system to take over controls.”14
The essence of the AI Maturity Model is the six maturity levels on which use cases can be classified. It also can be used to define the evolution of the ML-enabled computerized system and provides a first indication of the potential risk with regard to the selected ML application and use case design.14
The AI Maturity Model can also be used to define the evolution of the ML-enabled computerized system and provides a first indication of the potential risk with regard to the selected ML application and use case design.14
The maturity levels are as follows:
- Level I: System is used in parallel to the production process
- Level II: Non-AI applications
- Level III: Applications used in locked state
- Level IV: Autonomous with self-triggering learning—humans are in the loop, but they are used in combination with humans in operation who also control updates at all times
- Level V: Autonomous with self-triggering learning—without humans in the loop; human control relies solely on sampling after operations
- Level VI: Completely autonomous—optimizing either toward a defined goal or a direct feedback loop
Based on the AI Maturity Assessment, we move forward toward a holistic approach to identify potential GxP hazards as part of the ML risk and control framework. The maturity levels of the AI Maturity Model (i.e., clustering of autonomy and control design levels) should be mapped against the potential risk impact. The risk impact in terms of data integrity, product quality, and patient safety relates to the potential to impact the patient. The risk impact can be used to group applications:
- Indirect impact only
- Direct impact on GxP processes but no direct impact on patient safety
- Direct impact on patient safety via drug release
- Direct and immediate impact
On the far-left side of the risk impact matrix (see Figure 4), we find applications with indirect impact on product quality and data integrity. Examples are AI applications supporting post-marketing surveillance analysis or prioritization of complaints.
The second group comprises applications with direct impact on GxP processes, product quality of starting materials, or intermediate products, but no direct impact on patient safety. Examples are applications defining the right time for harvesting during the upstream process of cell growth. The chromatography example previously described would fall into this category.
The next group is made of applications involved in the production of the final pharmaceutical product, or the control and final approval by the drug release instance—for instance, the qualified person in the European Union and the quality department in the US. Applications in the last group, such as software-as-a-medical-device or software-in-a-medical-device, have a direct and immediate impact on patient safety. Based on the AI maturity level and the risk impact, the hazard impact can be defined.
The hazard impact—depicted in Figure 4 in green (low), orange (medium), red (high), and dark red (very high)—is the first level of risk assessment of an AI application in this risk and control framework. It should be noted that gray in the picture does not mean that no risk is associated, but that the risk is not ML specific. As illustrated in the following sections, the hazard impact is a central lever to be reviewed during the implementation. It will help determine whether the overall risk is acceptable for the AI system and to fine-tune it according to the risk appetite of the company, as illustrated in later sections.

In terms of the chromatography example, the hazard impact is determined to below. The application is categorized as level III on AI maturity because the application is used in a locked state: the model is only trained once and only retrained and revalidated on an individual basis. Furthermore, from a control design perspective, the application switches the fractions that can immediately be revoked by the applicant. From a risk impact perspective, the example has a direct impact on the GxP process and product quality but no direct impact on patient safety because a multitude of quality control measures follow.
Risk Assessment
Key to the risk control process is a holistic view on risks potentially affecting product quality or patient safety. Only if risks are identified can adequate risk control measures and monitoring procedures be defined—a “blind spot” might have an immediate impact on the quality objectives. However, ML-enabled applications are typically used in an environment of complexity. Often, a primary reason to opt for such a solution is to draw insights from complex input data and leverage complex algorithms consisting of various model layers and large parameter sets.
In addition, quality measures are statistical figures, which introduce an additional layer of uncertainty from a risk control perspective. Therefore, we deem a structured approach to the risk identification necessary, leveraging process understanding of how ML-enabled solutions are constructed and critical thinking reflecting on the specific use case.
GAMP 5® Second Edition10 introduced a life cycle model for the development of a ML subsystem in its Appendix D11. This life cycle consists of three major phases:
- Concept phase: The intended use is described by means of business, functional, and processual considerations. In addition, the availability and quality of data for training and productive operation are assessed.
- Project phase: Uses an iterative approach for data, models, and their hyperparameters, as well as model evaluation, to then shift toward a verification, acceptance, and release step. The iterative nature of this process reflects a growing understanding for data, processes, and the intended use. Subsequently, the verification and acceptance steps are designed to provide evidence as to whether quality objectives are met to prepare for a robust release.
- Operation phase: The ML subsystem is integrated within the computerized system architecture and serves its intended use, often in combination with human operators (“human-AI team”). During this phase, continuous monitoring must be performed and may result in changes or modifications to the AI/ML subsystem.
Following this life cycle model, we identified eight hazard clusters that should be assessed from a risk control perspective to prepare for the next stage of defining risk control and mitigations measures (see Figure 5).
- 10

In the following, the hazard clusters are elaborated including their rationale and their relevance in the context of product quality and patient safety in a GxP environment. The rationale follows the observation that, due to the statistical training and evaluation mechanics, every data point carries importance for the overall model. For this reason, inaccuracies in early stages, such as the data set identification or during model selection, may propagate to the production environment, as the evaluation in the test stage includes statistical uncertainty on its own. The rationales are thereby supported by the following risk inventory examples.
1. Initial data set quality
The quality of the case data set is crucial for the expected performance in operations. This data set must be able to provide enough representative data to a) train models on the actual intended use and b) ascertain the model performance in the test stage. If the initial data quality is low, the iterative learning process may be compromised.
Examples:
- The chosen data set is not adequately representative for the real-world application (selection bias).
- Labels of data may be inaccurate, which yields inferior directions to the training procedures and evaluation via key performance indicators during testing.
- Inaccuracies in data transformation applications (“Extract-Transform-Load”) lead to a wrong case set for learning and testing.
- Data augmentation techniques to complement base data are implemented inaccurately, posing a risk to representativeness.
- Data augmentation techniques may be more customized to the data used for the model development (training, validation, and test stages), which could lead to inferior performance during operations.
- Insufficient or inaccurate harmonization of data may blur the training, validation, and evaluation process, which may yield an inferior model performance.
2. Data split
Data splitting is a crucial task for being able to adequately test the performance before production on a data set not yet used for model development. If the data split yields a test data set that is not representative for productive use, a performance drop may occur in the operations phase.
Example:
- During determination of the training, validation, and test data sets, the test data set is not adequately representative anymore, which may distort key performance indicators and the final acceptance decision; if positive, immediate risk for product quality emerges.
3. Model design
An inferior model design (e.g., with a suboptimal choice of the modeling approach) may yield a less accurate model; in conjunction with progressive quality objectives, this causes a necessary higher risk to product quality or patient safety.
Examples:
- Inferior choice of model yields suboptimal performance given data and use case.
- The intended use may not closely match the actual real-world application, causing downstream risks to later steps in the project and operation phase.
- Quality expectations are too optimistic, posing higher risks to product quality and patient safety.
During operations, it must be ensured that the human is in control, otherwise an immediate risk regarding product quality, and eventually patient safety, arises.
4. Model training
On a similar note, as for the model design, but on a more detailed level, the search for an optimal set of features or hyperparameters may be stopped too early, causing a higher risk to product quality or patient safety.
Examples:
- Inferior selection of data (feature engineering) yields suboptimal performance regarding the quality objective profile.
- Iterative fine-tuning is stopped too early, which yields a suboptimal performance in the actual process, and hence risk to product quality and patient safety.
- The algorithm itself carries inaccuracies, while mistakes are overlooked because of the model complexity, which adds the risk of unexpected behavior in the production phase.
- The modeling path, design decisions, and fine-tuning process are not adequately documented, which yields user and inspector acceptance risks (resulting in a non-GxP risk, but a regulatory risk).
5. Model evaluation
The model evaluation is the final acceptance test before production; bias in the evaluation may yield an acceptance decision that does not conform to the organization’s established risk appetite.
Examples:
- The test data is more benevolent to the model than in median cases —which may yield low performance in operations, and hence a wrong acceptance choice and immediate risk to product quality.
- Inaccuracies in the model integration (i.e., provisioning of data, extracting model results) distort model performance, posing the risk of a wrong acceptance decision.
- Evaluation routines include inaccuracies, posing a risk to life cycle decision-making.
6. Deployment and release
Throughout the training and fine-tuning process, a large set of models is estimated. The wrong model choice for deployment and release poses an immediate risk to product quality and patient safety in the operations phase.
Examples:
- Out of a selection of possible iterations in the development process, the wrong model is deployed, which yields a nonvalidated and a possibly inferior or inadequate model in production.
- The infrastructure may not be adequate to support productive use, given the complexity of the ML model and possible explainability add-ons. This in turn may cause delays in decision-making along the productive process, and hence a risk to product quality.
7. Data quality in operation
If data quality does not meet the expectations as per evidence generated by use of the test data set, a loss in performance must be expected. Depending on the chosen operating model, and the degree of autonomy, a direct impact due to the decisions of the ML-enabled application, or at least an indirect impact due to confusion of operators, is expected.
Examples:
- The distribution of real-world data may gradually shift as, for example, in areas with lower statistical performance. This may yield more false positive cases or larger errors, as specified in the quality objectives.
- External or internal data sources may change syntax or semantics during runtime, which might not be reflected in the model, and cause a drop in performance and a risk to product quality depending on the use case.
- When relying on data or models provided by third parties, this may not be available according to the service level agreements, which may introduce a risk to the model performance and, therefore, product quality.
8. Human interaction and monitoring
During operations, it must be ensured that the human is in control, otherwise an immediate risk regarding product quality, and eventually patient safety, arises. The effectiveness of risk mitigation measures and performance of the human-AI team crucially depends on the design of monitoring and human interfaces.
Hazard Cluster | Hazard | Implication | Rationale and Comments |
---|---|---|---|
Initial Data Quality | Insufficient data was provided for training and testing. | The trained model might lack generalization; this might not be identified in the test step due to ranges of the data input space not sufficiently covered. | If the necessary data has not yet been collected, it is best to first accumulate sufficient data to estimate a more robust model. The sufficiency of the data can only be determined by experiments and by means of process and subject matter understanding. |
Data Quality in Operation | Granularity of input time series data for productive use is less than expected. | If fewer data points are provided in the time series than expected, the accuracy of the prediction is insufficient, translating to a use of the model in a nonvalidated input space area. | This hazard is quite typical when comparing training and test data. Much effort may have been invested into assembling suitable input data, while input data quality considerations might not be feasible in the productive context. Therefore, the nature and expectations regarding input data have to be clearly defined and validated in the acceptance step. |
Data Quality in Operation | Real-world data covers areas in the input space that have not been represented in the training and test data set. | If the characteristics of some time series are not reflected in the training and test data set and with inadequate generalization of the model, the accuracy of the prediction may be insufficient in turn giving rise to product quality risks. | Whether this hazard indeed leads to product quality implications depends on various characteristics of the model and the input data, e.g., the model’s generalization capabilities or the number of violations and the distance of the input to statistical mass in the training and test data set. |
Table 1
Examples:
- Features to facilitate human-machine interaction may be insufficient even after acceptance in the evaluation stage, which yields inferior decision-making or loss of time, and hence introduces a risk to product quality.
- Limitations and control of the ML-enabled system may be insufficient, so that decisions are based on uncertain outcomes.
- The monitoring may include inaccuracies or blind spots, posing a risk model for life cycle decisions (e.g., retraining or model redesign), which may yield undesired drift and gradual loss of model accuracy.
- For online learning systems, model drifts can pose a risk to the performance and reliability of the model, hence a lack of control in the production process.
As an excerpt for the chromatography optimization use case, Table 1 shows selected examples of risks according to their hazard cluster.
All identified hazards must be analyzed and evaluated. There are several classic risk management methods from fishbone diagrams to failure mode and effects analysis (FMEA). For IT systems, FMEA or variations of FMEA, which do not rely on risk priority numbers, are commonly used across the industry.
When it comes to complex processes and processes with continuous expected improvement, classical methods like FMEA are getting to their limitations. With increased complexity, the documentation for FMEAs expands, which results in the loss of transparency. This is particularly true if risks from different processes need to be put into perspective. As for complex processes, it is likely that some parts have inherently higher impact than others. An example of this is the drug manufacturing process, in which certain steps (e.g., preparation steps) have an intrinsically higher impact on data integrity, product quality, and patient safety than others.
For the specific example of drug development evaluation, the RAMM model was developed. The original RAMM model combines the risk rating of process steps with the individual risks. Via color coding, the visualization of risks across process steps for diverse critical quality attributes (CQAs) is achieved, which is not possible with other models.
As part of the RAMM model, the CQAs are listed on the Y-axes. For each CQA, the relative importance is determined, which reflects the relative impact of the CQA on product quality and patient safety. On the X-axes, the process steps and the process parameter, or the respective process attribute, are listed.
For controlling the risk of AI applications in more detail, we propose an adapted version of the RAMM model that reflects the complexity of the ML development cycles and facilitates the risk review, which is particularly critical for continuously improving systems. It thereby leverages the hazard impact level of the risk initiation as a hazard impact factor (HIF) and the hazard clusters of the hazard identification. In addition, it uses the quality dimensions of the AI Governance and QA Framework, as outlined in “AI Governance and Quality Assurance Framework: Process Design,”15 for the risk evaluation.
fig 6
In the rows of the AI RAMM table (see Figure 6), the application- specific risks, which were defined in the hazard identification, are listed and sorted along the hazard clusters. Here, the quality dimensions defined in the AI Governance & QA Framework are used,15 providing a blueprint to derive measurable AI quality expectations based on the intended use.
This facilitates effective communication along the life cycle. Based on the specific needs and experience of the organization, the detailed assessment along the quality dimensions could be skipped and a simple evaluation of low, medium, or high risk could be performed.
The original RAMM model uses a statically defined relative importance of the CQA, but for AI, the hazard impact, which depends on the hazard level defined in the risk severity matrix, is used. For the hazard impact, the following numbers are used based on the respective hazard level: low (1), medium (3), high (9), very high (18).
During the risk evaluation with the respective stakeholders, the risks defined under the hazard clusters are rated according to the quality dimensions. For the individual score, the risk ratings—marked in gray, e.g., low (1), medium (3), high (9)—are weighted by the HIF per quality dimension. For the total score of a risk, the individual scores per quality dimension are summed. The total score per risk can be used to determine when a mitigation is required and to prioritize the implementation of mitigation measures for different risks.
In addition, the hazard cluster total score can be determined by the sum of the total scores. The hazard cluster total score can be used to evaluate the need for additional controls and prioritization based on the hazard clusters. In addition, the overall score—because it is leveled by the hazard impact—can be used to compare various ML-enabled systems from a risk portfolio approach. This structuring of the AI RAMM provides a compromise between detailed risk evaluation and derivation of comparable scores to prioritize adequate measures.
Risk Control
Based on the completed risk assessment, risk reduction measures can be defined that can be used to reduce the individual risk rating. Typical measures are testing activities, the implementation of additional procedural or technical controls (similar to FMEA-based risk mitigations), or the collection of additional training data. The risk reduction action thereby impacts the individual ratings of the risks according to the quality dimensions. For the implementation of additional controls, it should be considered that these can introduce new risks.
During interactive sessions with respective stakeholders, the RAMM can be run through various iterations until the overall risk is considered acceptable. During these iterations, the proposed color code proves to be a core strength of the RAMM model because it visually highlights individual risks and the effects of risk reduction measures.
For AI systems where the risks cannot be mitigated to an acceptable level via individual risk reduction measures, the sum of risks can be reduced by an adjustment of the AI maturity level. This leads to a reduction of the risk severity level and impacts the overall evaluation and total score.
The adjustment of the AI maturity level can be influenced by redefining the AI autonomy or the AI control design. A mitigation via the adjustment along the X-axes in the risk severity matrix is usually not possible because this directly depends on the use case. Taking the sum of these measures, the risks can be brought to an acceptable level to complete the risk control cycle.
Risk Review
During the risk review, the full power of the proposed RAMM model becomes apparent. For AI systems moving to greater autonomy and taking over design controls in highly critical areas, the governance and thereby control of the operational phase is the critical factor. The periodic review of risks, refinement, and extension is key to ensure GxP compliance and assure trustworthiness of the system.
Therefore, the periodic cycle of risk reviews depends on the AI Maturity Assessment. During these risk reviews, the RAMM model provides a powerful tool to evaluate observations during normal operations, the impact of potential changes to algorithms, and the impact to the continuous improvement cycles along the AI Maturity Model stages and how to move the process up the hierarchy.
Thus, the RAMM model can also be used to put risks of different AI applications into perspective and to determine what is acceptable for stakeholders and where redesigns should be considered. In addition, given this dynamic setting, insights from similar or adjacent ML-enabled computerized systems may be used to reconsider the risk and risk mitigation strategy.
For instance, if risks are mitigated to a higher degree by one computerized system with controls and mitigations, similar ideas might be used to strengthen the risk strategy of a second system. Hence, the risk review should be performed with a holistic approach, leveraging best practices, process understanding, forward-looking methodology, and critical thinking.
Looking to the future of AI applications in GxP environments, we see iterative risk management cycles as the key concept to enable the use of truly autonomous learning algorithms of AI maturity level VI in a GxP environment.
Conclusion
We compiled an industry-specific ML risk and control framework based on the quality risk management process in the ICH Q9(R1) guideline, leveraging four key concepts: the AI Maturity Model,14 GAMP 5® Second Edition [10], the AI Governance & QA Framework,15 and RAMM.16 To this end, we have adapted the RAMM model to facilitate the risk management process.
What we call the AI RAMM model offers two main benefits. First, the multilevel structure allows teams to address AI application-specific risks and their scoring and to define risk mitigation throughout implementation projects. Second, it visually highlights critical areas using easy-to-comprehend color codes, which facilitate risk reviews that gain even higher importance with increasing autonomy and as presentations during audits.
In addition, the AI RAMM model provides organizations with a tool to compare risks across different ML applications and hence foster sharing of ideas, practices, and critical thinking concepts. The approach promotes active learning about risks and enables effective risk monitoring. Our downstream process optimization use case demonstrates the effectiveness of the ML risk and control framework in handling the complexity of ML-related risks in line with the ICH Q9(R1) guideline, exemplifying the conceptual hazard clusters to structure and improve risk oversight.
In conclusion, we can enhance the acceptance of AI usage in regulated areas of the industry by facilitating effective and efficient risk management of AI applications, possibly even in situations when dealing with dynamic online learning and ML operating models. Finally, the prioritization of risk mitigation activities that can yield maximum impact and provide feedback for subsequent risk management iterations are likely to enhance the quality of AI applications and related processes.
Acknowledgments
The authors would like to thank the ISPE D/A/CH (Germany, Austria, and Switzerland) Affiliate subcommittee on AI Validation and ISPE GAMP® Global Software Automation and Artificial Intelligence Special Interest Group (SIG) for their support on the creation of this ML risk and control framework. In particular, we would like to thank Laila Bekhet, Karl Laukenmann, Stefan Münch, Arif Rahman, Nagoor Shareef Shaik, and Michelle Vuolo for their review of this article and their fruitful discussions with the authors throughout the writing process.
- 15 a b c Altrabsheh, E., M. Heitmann, FRM, and A. Lochbronner. “AI Governance and QA Framework: AI Governance Process Design.” Pharmaceutical Engineering July/August 2022. https://ispe. org/pharmaceutical-engineering/july-august-2022/ai-governance-and-qa-framework-aigovernance- process
- 14
- 16Brindle, A., S. Davy, D. Tiffany, and C. Watts. “Risk Analysis and Mitigation Matrix (RAMM) – A Risk Tool for Quality Management.” Pharmaceutical Engineering January/February 2012. https://ispe.org/pharmaceutical-engineering/january-february-2012