Front-End Design of Personalized Medicine Facilities

The commercialization of personalized medicine has ushered in demand for a new type of facility—personalized medicine facilities—which can produce thousands of small-scale batches per year. There are currently only a handful of these sites, but many more are in various stages of design and construction. Designing these personalized medicine facilities presents new challenges, and a different design approach is necessary. Cyclic scheduling can produce high-quality models rapidly and aid collaborative design. A focus on room and zone availability and movements can prevent bottlenecks in corridors, gowning, and airlocks to streamline production.
Personalized Medicine Facilities and Bulk Drug Substance Production
The industry’s collective understanding of personalized medicine facilities is relatively immature, especially when compared to more established platforms for bulk drug substance production. Examples of more established platforms include, but are not limited to, small-molecule chemical active pharmaceutical ingredient (API), monoclonal antibody (mAb), and bacterial fermentation. The processes employed to make personalized therapeutics are also relatively immature, and we see a good deal of flux in process development during design.
CAR T Facilities
Of particular interest are autologous chimeric antigen receptor (CAR) T cell therapies. As of January 2024, there are only six such therapeutics approved by the US Food and Drug Administration (FDA).1 Accordingly, there are only a small number of facilities producing these therapies at scale. A large-scale facility would typically be capable of producing 4,000–10,000 patient batches per year.
The focus of this article is on production-scale CAR T facilities, which produce at least several thousand patient batches per year.
We have completed several front-end designs for large-scale CAR T facilities in recent years. From our experience, we have observed that such facili-ties require a different design approach than bulk API facilities. In this article, we outline these differences and identify some common pitfalls and key design considerations. Many of the issues relate to the scaled-out nature of production and are equally applicable to other similarly sized gene therapy facilities operating on other modalities.
We have also encountered challenges in creating scheduling models for these facilities using currently available software. In response to this, we have developed a proprietary approach for rapid generation of production scheduling simulations, which we outline in detail.
Design Approach
The main high-level difference between the production of bulk drug substances and personalized medicine is in scale, specifically scale-up vs. scale-out. Bulk drug substance production requires large equipment, with reactors in the thousands, or tens of thousands, of liters and with 10 or more batches per year. Personalized medicine produces small-scale batches, typically no more than a few hundred grams, but it produces perhaps ten thousand such batches per year.
With the scaled-up nature of bulk drug substance production, the focus is on equipment sizing, and the general approach is that we design around the needs of the core process equipment. With personalized medicine, considerations such as personnel and material flow, which are usually peripheral to the design of a bulk facility, are of critical importance. The two approaches are contrasted in the following section.
Bulk Drug Substance Approach: Focus on Equipment
As process engineers, we have developed mature procedures for facility design centered on our many years of experience with bulk API facilities. With small-molecule chemical API facilities, designs often accommodate many different short campaigns to make intermediates, with any given reactor used for several different reactions. The complete process to make the drug substance is generally broken up into many subprocesses, with the intermediates bulked and stored in between.
These facility designs often need to account for multiple intermediate production recipes, with a strong focus on the requirements for campaign changeover and line clearance. A great deal of design focus is also centered on utilities, solvent storage, emissions, and waste.
With bulk biologics (e.g., mAb and bacterial fermentation), the focus is generally centered on efficiency and throughput. Batches tend to be run straight through from vial thaw to drug substance formulation. Core equipment tends to be used once per batch. Design challenges revolve around the size and count of upstream trains, and the sizing of downstream equipment, with a focus on balancing equipment size against utilization. The sizing and scheduling of solution preparation is also a key consideration.
Core equipment vs. support equipment
It is important, at this point, to differentiate between core equipment and support equipment. Core equipment is the equipment that is used in the core process and typically has physical contact with the work-in-progress batch. Support equipment comprises utilities, waste management, clean-in-place (CIP) skids, solution preparation and hold vessels, and tank farms.
With bulk facilities, the primary challenge during front-end design is to optimize the sizing and count of the core equipment to meet throughput requirements in an efficient manner. In general, the design of core equipment should not be constrained by any outside factors. Having done this, the design scope widens to encompass the design of support equipment, such as solution preparation and utilities.
In the front-end design of bulk facilities, labor and discrete movements are often not a key design consideration. In general, the core equipment has primacy, and the assumption is that adequate labor will be provided to ensure the process runs smoothly. Changes to labor requirements tend to have a minimal impact on cost and layout, being perhaps limited to some scaling of gowning areas.
Although bulk facilities do include discrete material movements, such as powder containers, movable equipment, and single-use consumables, it is unlikely that the facility will be bottlenecked by logistical concerns.
Risk and redundancy
A final design consideration is a philosophy on risk and redundancy. For bulk facilities, this is primarily an economic consideration: balancing the cost of providing redundancy against the probability and cost of a failed batch due to equipment unavailability or breakdown. In general, for bulk facilities, there tends not to be any redundant core equipment, unless a particular piece of equipment has many units in parallel (such as, occasionally, reactors and bioreactors) or has complex routine maintenance requirements (such as centrifuges). We would typically see some redundancy in clean utilities, solution preparation, and CIP skids.
Bulk drug substance facility design
Bulk drug substance facility design typically proceeds as follows. First, identify overarching design goals and degrees of freedom. Questions in this step can include:
- What are throughput requirements; are they specified in terms of batch quantity or volume or mass of product?
- Are we designing for one or more products?
- Do we need to accommodate process variability, e.g., titer ranges?
- Is a phased design required to meet a demand ramp?
- Are key equipment sizes and counts fixed, or are they a degree of freedom?
Next, prepare parameterized mass balances to size primary equipment. The mass balance should be easy to scale if, e.g., batch size or equipment size is varied. Prepare production schedules to validate and optimize sizing of core equipment. This can be challenging if complex changeover scenarios are a feature, but the task is usually straightforward for single-product facilities because core equipment should not wait for anything.
Next, use the production schedule and mass balance to size utilities and support equipment. This is significantly more challenging than the previous step because non-core equipment often experiences competition from multiple sources.
Finally, we consider support equipment. Consider support vessels first (e.g., solution preparation), followed by cleaning systems, then clean and black utilities.
Personalized Medicine Approach: Focus on Flow
Large-scale personalized medicine production facilities present very different design challenges. Adopting an equipment-focused approach can trip up designers due to the more profound impact of personnel and material flows.
These facilities typically produce thousands of batches per year, perhaps two orders of magnitude more than a typical bulk biologics or chemical API facility. The processes involved are currently very labor-intensive and a typical batch may require over 100 individual consumables, typically packed into kits. Each batch produces perhaps a dozen quality control (QC) samples, giving tens or hundreds of thousands of samples per year. It is clear from the scale of these discrete movements that they will have a much greater impact on facility design than in a bulk facility that makes a few batches per week and relies mainly on piped transfers.
Facility layout
In bulk drug substance facilities, the size and layout of the facility is primarily driven by equipment requirements and adjacencies. In personalized medicine facilities, we still need to accommodate the process equipment, but we are often dealing with a mix of benchtop equipment, biosafety cabi-nets, gloveboxes, and stackable incubators. Of equal importance in a room is a focus on headcount, ergonomics, and staging space.
Comparison | Bulk Drug Substance Facility | Personalized Medicine Facility |
---|---|---|
Scale | Large process equipment, relatively small count of core process equipment | Mainly benchtop equipment, high degree of scale-out with 10 or more parallel equipment items |
Material Transfers | Mainly piped | Mainly discrete movements |
Throughput | 10–100 batches per year | 1,000–10,000 batches per year |
QC | Hundreds of tests per year | Thousands or tens of thousands of tests per year |
Key Considerations | Equipment sizing, solution preparation, utilities, solvents, and waste | Equipment and suite counts; movement of kits, personnel, samples, and product; and ability to clean rooms and corridors |
In personalized medicine facilities, corridors, gowning, and airlocks can become bottlenecks in the process. The ability to deep-clean spaces with sporicidal agents needs to be considered early in the design, as this may prevent personnel from accessing rooms and corridors for several hours a week. If these features are not considered thoroughly throughout the design process, a facility can become bottlenecked by its infrastructure, rather than its core process equipment.
One key degree of freedom, when designing for scaled-out production, is the tradeoff between room size and room quantity. Fewer, larger rooms lead to a more space-efficient design but make it difficult to carry out cleaning. Using an increased number of smaller rooms reduces the likelihood of cross-contamination and reduces the risk of equipment, such as air handling units, failing. However, this strategy also requires more space and complexity and can hamper flexibility. A balance must be struck to arrive at a reasonable design.
Material movements
The impact of discrete material movements can have a profound effect on layout and facility size. Larger rooms may require multiple material air locks (MALs) or personnel air locks (PALs) so that material and personnel movements do not become a production bottleneck. The space required for kitting and movement from non-graded space into the suite can be substantial. As a result, we require data on the scheduling of material movements early in the design process. This usually comes in the form of a bill of materials (BOM), plus a separate BOM for discrete waste streams.
Personalized medicine facility design
Personalized medicine facility design typically proceeds as follows. First, identify overarching design goals and degrees of freedom. Questions in this step can include:
- What are the throughput requirements?
- Do we need to accommodate process variability?
- Is a phased design required to meet a demand ramp?
- What are redundancy requirements (at room, suite, workstation, and equipment levels)?
Next, a BOM and waste BOM should be drawn up (a detailed mass balance is not typically required). Prepare production schedules to validate and op-timize count of suites and equipment. Several options may be investigated here, so a structured approach should be taken to allow for multiple models to be maintained simultaneously with minimal duplication of data.
Then, add detail to the production schedule, such as material movements and personnel requirements. Consider room and corridor cleaning requirements and the capacity of MALs, PALs, rooms, corridors, and gowning. Some iteration will be required to arrive at one or more viable solutions.
Capacity Modeling
To prove a facility design is viable, a capacity model is required. The aim of a capacity model is to demonstrate how the required throughput can be scheduled on equipment in the facility while ensuring that demands for material movements, labor, and cleaning can be reasonably met. We have considered developing production models using several methods. We found that recipe-based scheduling software is too slow to solve problems, and that discrete-event simulations are too slow to build and their complexity is a barrier to collaboration.
Evaluating Existing Techniques
Recipe-based scheduling software is ubiquitous in the pharmaceutical industry and is widely used to aid in the design of bulk substance production facilities. We have found that this type of software does not perform well when there are many simultaneous batches in process at a given time. Take, for example, a large-scale CAR T facility. If it produces 10,000 batches per year, and a batch takes two weeks to produce, there will be over 400 batches in production at any given time. The number of batches to be scheduled will be even greater than this, 500 or 600 to give a few days of fully occupied steady-state production in the middle of the scheduled campaign.
Even with straightforward models, with zero degrees of freedom, we are seeing solution times running into hours, making this approach intractable for rapid design development. Furthermore, the clash-resolution algorithms in this type of software are of limited efficacy when batches overlap considerably. This is a limitation of the technique, rather than the software; the mathematical complexity of this form of scheduling tends to make solution times grow exponentially with model size.2
Discrete-event simulation (DES) can also be used to model large-scale personalized medicine facilities.3 DESs are structurally very simple:4
- A clock starts at time zero and keeps track of current time.
- An event in the model consists of an action and a time.
- A data structure called a “priority queue” keeps track of future events and keeps them in order.
- The model is run by continuously popping the next event from the head of the queue, setting the clock time to the event time, and then executing the event actions. This is repeated until the queue is empty.
- Note that event actions often involve the creation of more events. For example, if the model executes a “start incubation” action, this may add a “finish incubation” event to the queue to be executed in the future.
DES solution times tend to scale linearly with problem size and therefore can deal well with large models. DES software can be used to create incredibly detailed models of personalized medicine facilities, including 2D and 3D dynamic models. Such models tend to be complex to build, requiring a good deal of decision-making about movements and operating rules. Although the models they produce can be powerful tools for optimizing and understanding these facilities, they remain too slow and complex to build (rather than solve) and are accordingly not a good fit for short-duration front-end design.
Another important factor is the relatability of the model. Is it in a format that the client can interact with and understand? Is it simple enough to explain and defend in a short presentation? Is it easy to read the input and output data? Because DES is quite unstructured, it can be difficult to explain and characterize a model. It is difficult for a client to see all the data in the model and to understand the rules implemented within.
During front-end design, when there is rapid churn, it is critical to have a model that can be modified in near real time, so that both the designer and the client can coalesce on a workable design. It is our view that DES would represent over-simulation at this phase of design, requiring a great num-ber of philosophical decisions to be made regarding movements, staging, and interactions. DES does remain a valid tool for detailed design or for modeling an existing facility in operation.
A Cyclic Approach to Scheduling
For a large-scale personalized medicine facility, we are generally considering 24/7 production and a few dozen batches per day. It is reasonable to assume, therefore, that every day is scheduled identically (with adequate downtime each day for cleaning and routine maintenance). Furthermore, we can usually assume that the nth batch on any given day will always use the same equipment.
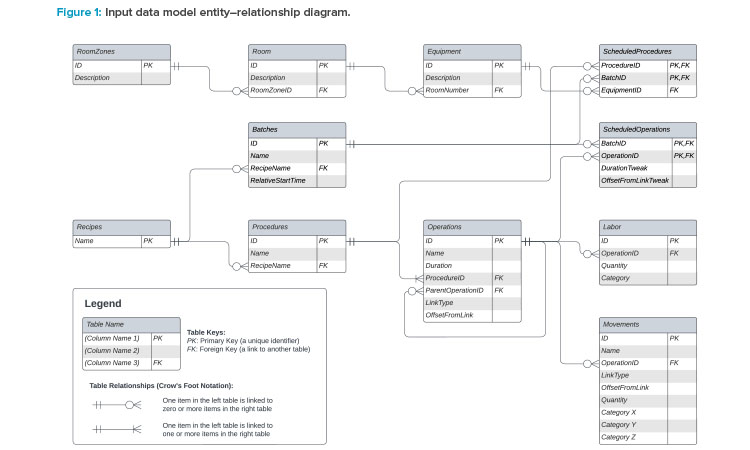
Finally, we are usually in the situation where all process procedures take less than a day (apart from incubation). This allows us to make an important simplification. Instead of looking at a long scheduling horizon of several weeks and hundreds of batches, we need only consider a 24-hour window. This window captures steady-state production, with the assumption that the 24 hours on either side of this window will be identical, and so on.
Borrowing from recipe-based scheduling software, we can define the production recipe, and its durations, but then use modular arithmetic to wrap the schedule around on itself and just focus on this short, repeating window. Note that in principle, the approach could be extended to consider longer or shorter repeating periods (e.g., weeks), with an accompanying increase or decrease in model size.
The Input Data Model
To develop a capacity model on the preceding premise, we must first create an input data model to make sense of data requirements. Consider this to be like a database, with tables of related data. (Indeed, a database is well-suited to capture such data, but it could more easily be built using tables in a spreadsheet or plaintext files.) A high-level data model is shown in Figure 1. The terminology used in this model is based on the ISA-88 standard.5
A summary of the tables is given next:
Equipment
This can represent a single piece of tagged equipment (e.g., a cell counter) or a collection of equipment (e.g., a workstation including a tube welder, some process equipment, and a cell counter).
Rooms and room zones
These constructs allow us to specify where equipment is, allowing us to plan idle time for cleaning. By grouping rooms into zones, we can see when the entire zone is available to be cleaned.
Recipes
A recipe is the top-level of a set of instructions for how to make a single batch. In a typical model, we may just have a single production recipe (which is run many times per day) and a daily-task recipe (e.g., for daily setup or restocking tasks).
Procedures
Each recipe consists of many procedures (e.g., “activation” or “transduction”) that involve constraining a single piece of equipment for their duration.
Operations
These are the building blocks of procedures (e.g., “setup,” “mix,” “teardown”) and can be linked to other operations in various ways. Durations are defined at the operation level. The scheduling of a procedure is calculated from the scheduling of its constituent operations.
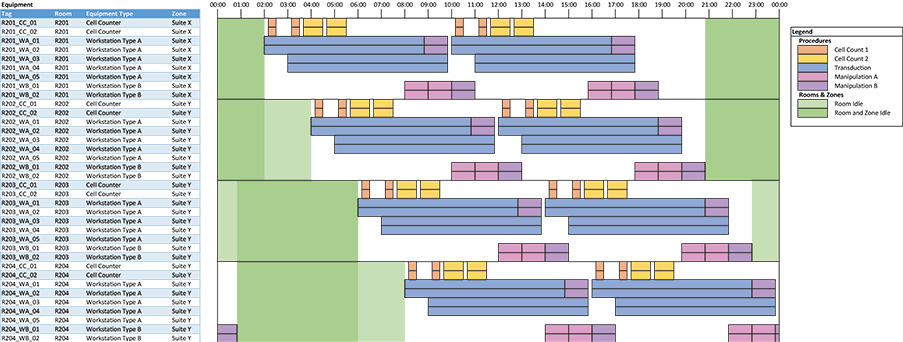
Labor
Labor requirements are attached to operations. More than one category of labor may be required for a given operation, and the quantity of each category can be specified.
Movements
Movements are attached to operations and are assumed to be instantaneous. This table is used to track the movement of work-in-progress batches, samples, and kits from the point of view of process demand (i.e., when the process needs a kit or emits a sample). During front-end design, it is unlikely there will be sufficient time to work thorough exactly how each movement is staged. It should be sufficient to track when the movements occur from the point of view of the process, and to consider, for example, hourly averages by room when looking at MAL or corridor utilization.
Batches
Batches are instances of the recipe, scheduled to start at particular times during the 24-hour window, with the assumption that similar batches start at the same time and use the same equipment every day. Our first important degree of freedom is in how batches are scheduled and staggered throughout the day.
Scheduled procedures
This table specifies the equipment required to carry out a particular procedure in a particular daily batch, forming the second major degree of freedom in the model.
Scheduled operations
This table is used to tweak the scheduling of particular batches by, for example, delaying or pulling forward tasks or modifying the duration of tasks by some delta. Ideally, every batch will be scheduled identically relative to its batch start, but occasionally it is useful to have this additional degree of freedom to resolve minor clashes. Incubation durations are usually defined as a range, so some of this rangeability can be used to resolve short-duration clashes.
From the input data model, it should be clear that we have all the data necessary to calculate the exact timing of every procedure, labor requirement, and movement requirement in our repeating 24-hour window. Although we are not going to derive the sort of clash-resolution algorithms found in recipe-based scheduling software, we have found that this is unnecessary.
In any case, such software struggles to resolve clashes in models of this scale. It is possible to create a model in a spreadsheet (without recourse to any macros or scripts) that can fully describe the schedule and highlight clashes visually and calculate almost instantaneously. This allows us to resolve scheduling clashes manually and rapidly by assigning a procedure to different equipment or by delaying or pulling forward an operation.
Outputs
The most important output is a plot showing a clear equipment schedule (for the 24-hour repeating window). This is not something that is possible to do natively with the limited plotting capabilities of spreadsheet software, but we have found an approach using conditional formatting that allows us to create clear plots. This is done by discretizing equipment occupancy into, for example, 5- or 10-minute blocks and coloring in cells (using conditional formatting) to compose the plot. See the example in Figure 2.
In the equipment schedule plot example, each row represents tagged equipment. Colored blocks indicate procedures occurring in equipment. The plot can be filtered by room or equipment type. The available time for cleaning is shown in green; lighter green blocks indicate the room is free and darker green blocks indicate that the room and zone are both free. This allows us to identify blocks of time where deep cleans of corridors can be scheduled.
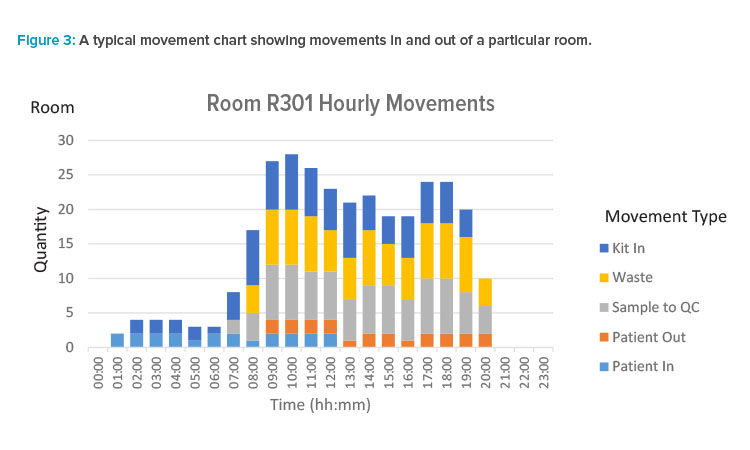
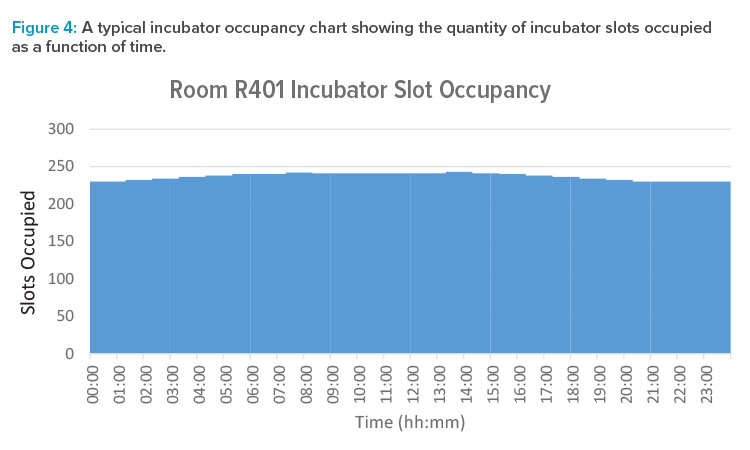
In addition to the scheduling of equipment, the resolved model gives us data on personnel and movement scheduling. We can generate useful graphical outputs from this, such as pivot charts that allow us to see labor requirements by room and/or labor category. Labor demand is linked to operations. Based on the resolved schedule, we can calculate the start and finish times for each use of labor. We can then trend labor demand over time. With labor requirements, we are typically interested in real-time demand to allow us to identify, for example, peaks in room occupancy.
We can also generate similar charts showing movements in and out of rooms by category. Movements are assumed to be instantaneous in the model. Based on the resolved schedule, the time for each movement occurrence is known. With movements, we typically sample over longer periods for the purposes of plotting. For example, we may be interested in the movement counts in each hour, broken down by category, for a particular room.
This longer interval is more appropriate, as the schedule only tells us when the process consumes or emits a material, and we are not at the stage in the design where we are ready to reason about how exactly kits and waste are ordered, staged, or removed. What we are aiming for is an indication of busy periods in MALs so that we can decide qualitatively if there is broadly sufficient MAL capacity and staging to allow some movements in to occur ahead of time and some movements out to be delayed. A typical movement chart is shown in Figure 3.
We can treat long-duration procedures such as multiday incubation as a special case. Within a room, we count total incubator slot occupancy, rather than trying to model each individual slot within the banks of incubators. This can be displayed as a bar chart or step function plot, as per Figure 4, which shows the quantity of slots occupied as a function of time.
Benefits of Cyclic Scheduling
The following list includes some of the many benefits of cyclic scheduling:
A well-defined input data model can drive the handover of information from the client in a clear manner.Each table in the input data model can be easily implemented in a spreadsheet, giving both the designer and client a resource that is human-readable and easy to check.The model resolves in seconds and highlights clashes, enabling rapid design development. (We have found that it is responsive enough to allow updates in real time during design workshops.)The equipment schedule plot provides a clear tool to visualize the operation of the facility.The ability to visualize room and zone availability aids the development of a cleaning philosophy.The ability to plot daily trends in movements and headcount allows constraints in labor, MALs, and kitting to be factored in earlier during the design process.
Conclusion
We have highlighted the differences between designing a bulk drug substance production facility and a large-scale personalized medicine facility. In particular, designers need to account for the large number of discrete movements and must allow adequate time in the production schedule for room and corridor cleaning.
Faced with the challenge of designing scaled-out personalized medicine facilities against tight front-end design timelines, we have found that existing methods are a bottleneck to the design process. By developing a modeling philosophy around cyclic scheduling, we can develop high-quality models rapidly. These models are in a format that is easy to comprehend, without stakeholders needing familiarity with complex software packages. This approach aids collaborative design. By focusing on room and zone availability and movements as part of our design, we can preempt bottlenecks particular to personalized medicine facilities.
Acknowledgements
The authors would like to thank the ISPE PQLI ICH Q12 Technical Team for their support.