Artificial Intelligence Governance in GxP Environments

Artificial intelligence (AI) is used by pharmaceutical and biotech companies, providing support from drug discovery through manufacturing. The nature of AI and concerns of bias, privacy, transparency, and security in a regulated industry necessitate a governance framework to ensure concerns are controlled using “guardrails.” These guardrails ensure the quality, privacy, and security of data used in AI applications. This article provides a recommended approach to implementing guardrails through several policies and procedures and discusses AI governance, which defines data ownership, consent, and access policies and procedures.
This article provides points to consider for those implementing AI in a GxP environment. At a high level, these include governance for AI, as well as for machine learning operations (MLOps). MLOps is a set of practices that automate and simplify machine learning (ML) workflows and deployments
Definitions for AI, generative AI, ML, and deep learning (DL) can be found in ISO/IEC Standard 22989, “Information Technology — Artificial Intelligence — Artificial Intelligence Concepts and Terminology”
AI USE: FROM DRUG DESIGN TO DISTRIBUTION
AI is used throughout drug discovery, drug product development, clinical development, manufacturing, and distribution. Within drug discovery, drug design (e.g., target protein structure prediction) and drug screening (e.g., bioactivity prediction, toxicity prediction, and physicochemical property prediction) are impacted areas benefiting from AI
Clinical trial design and monitoring (e.g., subject enrollment/selection, patient drop out, and trial monitoring), manufacturing (e.g., automated manufacturing, personalized manufacturing, and correlating manufacturing errors to set parameters), and quality assurance/quality control (e.g., electronic lab notebooks) use AI to improve decision-making as well
In post-marketing, AI could also be used to analyze data to predict a new indication or usage of the drug by using real-world data collected after the drug was marketed and/or in combination with the pre-marketing data. The drug’s new indication or usage may extend the drug’s patent expiration date.
AI-associated processes, such as data collection, processing, analysis, storage, and utilization of algorithms to derive decisions, would require guardrails to assure data quality and integrity so that the results are accurate, reliable, trustworthy, and explainable.
AI GOVERNANCE
AI governance is a system of laws, regulations, policies, controls, frameworks, standards (e.g., ISO/IEC Standard 42001, “Information Technology Artificial Intelligence — Management System”), practices, and processes at international, national, and organizational levels to manage, regulate, and optimize software application development, deployment, and usage within an organization. AI governance allows AI-applicable technology stakeholders to manage, regulate, optimize, implement, and oversee the use of AI technology
AI governance could further set ethical principles by controlling how AI is developed, implemented, and used. Consistent, end-to-end AI governance would enhance internal capabilities through methodologies and tools that address critical ethical requirements such as accountability, fairness, privacy, transparency, and robustness.
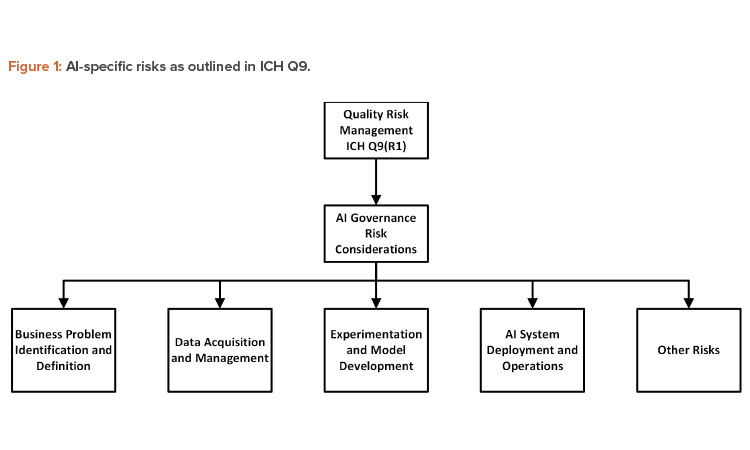
The recommended approach for AI governance throughout this document could be seamlessly integrated with ISPE GAMP® 5 Guide: A Risk-Based Approach to Compliant GxP Computerized Systems (Second Edition)
Quality Risk Management
Quality risk management (QRM) processes as per “ICH Harmonized Tripartite Guideline Q9: Quality Risk Management” (e.g., risk assessment, risk control, and risk review) must be implemented
The implementation and/or use of the policies and procedures noted throughout this article should be conducted considering a risk-based approach, with only a subset of the documents recommended being implemented for lower-risk systems. Overall risk should be determined by impact to patient, product, and data integrity.
A quality management system (QMS) in alignment with the Pharmaceutical Inspection Convention Pharmaceutical Inspection Co-Operation Scheme (PIC/S) “PIC/S Guide to Good Manufacturing Practice for Medicinal Products - Part I”
Risk Area | Topics for Consideration |
---|---|
Business problem identification and definition |
|
Data acquisition and management |
|
Experimentation and model development |
|
AI system deployment and operations |
|
Other risks |
|
AI GOVERNANCE POLICIES
Creating policies for an AI governance framework is essential to ensure that AI systems are developed, deployed, and managed responsibly and ethically. It ensures the systems are tested and validated for GxP intended use and comply with data governance and cybersecurity policies and regulations. The following are some key policies that organizations should consider implementing as part of their AI governance framework
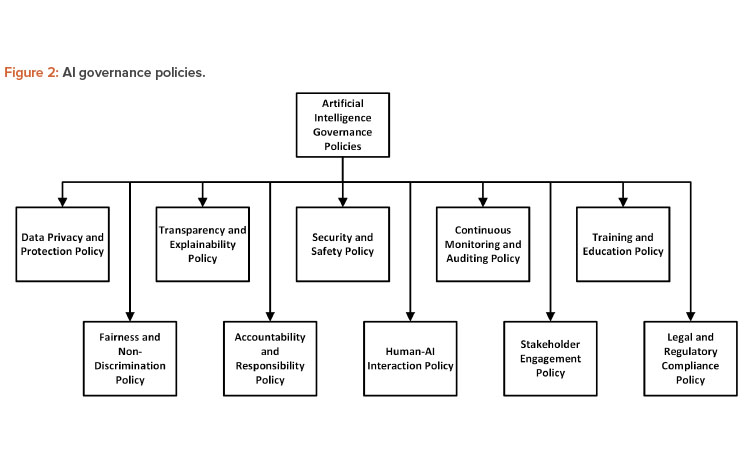
Data Privacy and Protection
Establish a policy for AI systems. Ensure compliance with data protection laws such as collecting, storing, and processing data used in the General Data Protection Regulation, California Consumer Privacy Act, and EU AI Act. Define obtaining informed consent, anonymizing data, and protecting sensitive information.
Fairness and Nondiscrimination
Develop a policy to prevent and mitigate bias and discrimination in AI systems. It includes conducting regular audits to identify and address sources of bias in data, algorithms, and models. Establish guidelines for fairness testing and validation.
Transparency and Explain ability
Create a policy to ensure that AI systems are transparent and explainable. Provide documentation and explanations of AI models, decision-making processes, and
outcomes. Make sure that stakeholders can understand and trust AI systems.
Accountability and Responsibility
Define clear roles and responsibilities for developing, deploying, and managing AI systems. Assign accountability for addressing issues such as bias, discrimination, and errors in AI systems. Establish procedures for reporting and addressing AI-related incidents.
Security and Safety
Implement a policy to ensure the security and safety of AI systems. Protect AI systems from unauthorized access, tampering, and attacks. Establish guidelines for secure coding practices, vulnerability assessments, and incident response.
Human–AI Interaction
Develop a policy for human–AI interaction, including guidelines for human oversight and intervention in AI systems. Define procedures for handling situations where AI systems provide incorrect or harmful recommendations.
Continuous Monitoring and Auditing
Create a policy for continuous monitoring and auditing of AI systems. Establish the tracking of the performance and impact of AI systems over time. Regularly review and update AI models to meet ethical and legal standards.
Stakeholder Engagement
Establish a policy for engaging with stakeholders, including employees, customers, regulators, and the public. Gather input and feedback on AI systems to ensure alignment with the values and needs of the community.
Training and Education
Implement a policy for training and education on AI ethics, best practices, and governance. Provide resources and support for employees and other stakeholders to build a culture of responsible AI.
Legal and Regulatory Compliance
Develop a policy to ensure AI systems comply with relevant laws and regulations. Establish conducting legal and regulatory assessments of AI systems.
AI GOVERNANCE FRAMEWORK PROCEDURES
Creating procedures for an AI governance framework is essential to operationalizing the policies established in the framework. This section includes essential procedures that organizations should consider implementing as part of their AI governance framework
Data Management
Establish a procedure for collecting, storing, and processing GxP data used in AI systems. Define steps for data cleaning, validation, and transformation. Ensure that data is representative, unbiased, and of high quality.
Bias Detection and Mitigation
Develop a procedure for identifying and addressing bias in AI systems. Define steps for conducting fairness audits, analyzing sources of bias, and implementing bias mitigation techniques.
Model Development and Validation
Create a procedure for developing and validating AI models. Define feature selection, model training, hyperparameter tuning, and model evaluation steps. Ensure that models are robust, accurate, and generalizable. Continuous training and continuous testing (or retesting) should be considered where the addition of data, fine-tuning of the algorithm(s), and/or retraining of the model occur. In addition, new patterns of bias should be investigated.
Transparency and Explainability
Establish a procedure for providing transparency and explainability in AI systems. Define steps for generating explanations, visualizations, and documentation of AI models and decision-making processes. The verification of AI-generated content would need to be considered by cross-referencing the infor-mation with credible sources; verifying facts, statistics, and claims against multiple trustworthy references; monitoring the AI’s performance and reviewing its output regularly; and assessing the generated content for potential biases, errors, or inconsistencies
Security and Safety
Implement a procedure for ensuring the security and safety of AI systems. Define steps for conducting vulnerability assessments, implementing secure coding practices, and responding to security incidents.
Human–AI Interaction
Develop a procedure for human–AI interaction, including human oversight and intervention in AI systems. Define steps for handling situations where AI systems provide incorrect or harmful recommendations.
Monitoring and Auditing
Create a procedure for continuous monitoring and auditing of AI systems. Define steps for tracking performance metrics, conducting impact assessments, and updating AI models.
Stakeholder Engagement
Establish a procedure for engaging with stakeholders, including employees, customers, regulators, and the public. Define steps for gathering input, addressing concerns, and incorporating feedback into AI systems.
Training and Education
Implement a procedure for providing training and education on AI ethics, best practices, and governance. Define steps for conducting training sessions, providing resources, and assessing knowledge and skills.
Incident Response
Develop a procedure for responding to AI-related incidents, such as bias, discrimination, and errors. Define steps for reporting incidents, conducting investigations, and implementing corrective actions.
Legal and Regulatory Compliance
Create a procedure for ensuring compliance with relevant laws and regulations. Define steps for conducting legal and regulatory assessments, obtaining approvals, and maintaining documentation. It’s recommended to add a compliance issue escalation process (including responsibilities) to this procedure.
MLOps PROCEDURES
MLOps is a set of practices that unifies ML system development and operations (Ops). It aims to automate the end-to-end ML life cycle, ensuring faster experimentation, deployment, reproducibility, and monitoring. For a robust MLOps framework, several procedures should be established
Version Control
Use version control systems for code, data, and model artifacts, and to ensure traceability of changes and facilitate collaboration among team members.
Data Management
This procedure should define protocols for data collection, storage, preprocessing, and validation; implement data versioning to track changes and ensure reproducibility; and monitor data for drifts or anomalies that might affect model performance.
Model Development and Validation
This procedure should establish coding and architecture standards for model development, implement automated testing for model validation, and use techniques like cross-validation to assess model performance. It should be used in addition to computer validation (e.g., GAMP® 5, Second Edition expectations).
Continuous Integration and Continuous Deployment (CI/CD)
This procedure should automate the integration of new code, data, or model changes. It should ensure automated testing at each integration step and automate the
deployment of validated models to production.
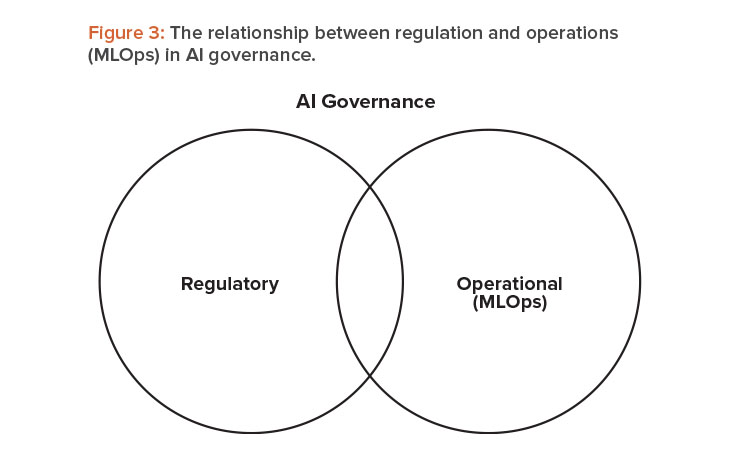
Model Monitoring and Logging
This procedure should monitor deployed models for performance degradation or drift and log model predictions, inputs, and anomalies for traceability and debugging.
Model Retraining and Fine-Tuning
This procedure should define criteria for when and how models should be retrained and automate the retraining process using updated data or when performance drops below a threshold.
Experiment Tracking and Management
This procedure should use tools to track and manage multiple experiments, hyperparameters, and results. It should also ensure reproducibility by logging experiment details and outcomes.
Infrastructure and Environment Management
This procedure should define protocols for provisioning, managing, and scaling infrastructure resources. It should also ensure consistency in development, testing, and production environments using containerization or virtualization.
Model Interpretability and Explainability
This procedure should implement tools and practices to interpret and explain model predictions and should ensure stakeholders understand model decisions, especially in
critical applications.
Model Security and Compliance
This procedure should establish protocols for model security, including access controls and encryption, and ensure compliance with data privacy regulations and industry-specific standards.
Feedback Loop
This procedure should implement mechanisms to gather feedback from end-users or system interactions and then use feedback to improve models and address any issues or concerns.
Rollback and Disaster Recovery
This procedure should define protocols for rolling back deployments in case of failures or issues and ensure backup and recovery mechanisms for data, code, and models.
Collaboration and Communication
This procedure should facilitate collaboration among data scientists, ML engineers, DevOps, and other stakeholders. It should ensure clear communication channels for updates, issues, or changes in the MLOps pipeline.
MLOPS AND AI GOVERNANCE RELATIONSHIP
MLOps and AI governance are essential for responsible, effective AI development and deployment. They are closely related and complement each other but focus on different aspects of the AI life cycle. The following sections explain how they are related
Relationship
MLOps focuses on the operational aspects of ML, including model development, deployment, monitoring, and maintenance. It aims to automate and streamline the ML life cycle, improve collaboration between teams, and ensure the reproducibility and scalability of models.
AI governance focuses on AI’s ethical, legal, and social aspects, including fairness, transparency, accountability, and privacy. It aims to establish guidelines, policies, and procedures for the responsible and ethical development, deployment, and management of AI systems. This incorporates the regulatory expectation for thorough and reliable AI governance.
MLOps and AI governance overlap in model monitoring, validation, and documentation. MLOps provides the tools and practices for implementing these activities, whereas AI governance provides the principles and standards for guiding these activities. MLOps and AI governance emphasize the importance of stakeholder engagement, continuous improvement, and compliance with laws and regulations. They work together to ensure that AI systems are aligned with the values and needs of the community.
Implementation
Practices of AI governance and MLOps are considered together when developing the overall AI paradigm. This is to ensure governance is practical and implementable, and that complete and operational paradigms are capable of conforming to governance.
AI governance can be integrated into MLOps by incorporating ethical considerations into operational workflows. For example, MLOps can include bias detection and mitigation steps in model development pipelines, and model monitoring systems can track fairness and performance metrics.
Some examples (though not an exhaustive list) of procedural and technical controls, or guardrails, include:
- For US FDA–regulated drug companies: “21 CFR Part 11, Data Integrity and Compliance with Drug CGMP - Questions and Answers,” GAMP® 5, Second Edition, and associated ISPE Good Practice Guides
- For EU-regulated drug companies: “EudraLex Volume 4 Annex 11”
- For UK-regulated drug companies: “Medicines & Healthcare Products Regulatory Agency (MHRA) ‘GXP’ Data Integrity Guidance and Definitions”
Benefits
MLOps and AI governance provide a comprehensive approach to AI development and deployment. MLOps ensures that AI systems are efficient, reliable, and scalable, whereas AI governance ensures that AI systems are ethical, transparent, and accountable. By combining MLOps and AI governance, organizations can achieve operational excellence and ethical responsibility in AI. It can lead to better AI outcomes, more significant AI impact, and higher AI trust.
MLOps and AI governance are closely related and complementary to the AI life cycle
CONCLUSION
Effective AI governance implementation requires collaboration across different departments and levels of an organization. It involves establishing a governance framework, defining roles and responsibilities, implementing technology solutions, and fostering an AI-driven culture. By adhering to AI governance principles, organizations can maximize the value of their AI assets while mitigating risks associated with poor AI quality. This includes escalation of GxP compliance issues that could impact quality attributes and data integrity principles and their resolution.