AI Governance and QA Framework: AI Governance Process Design
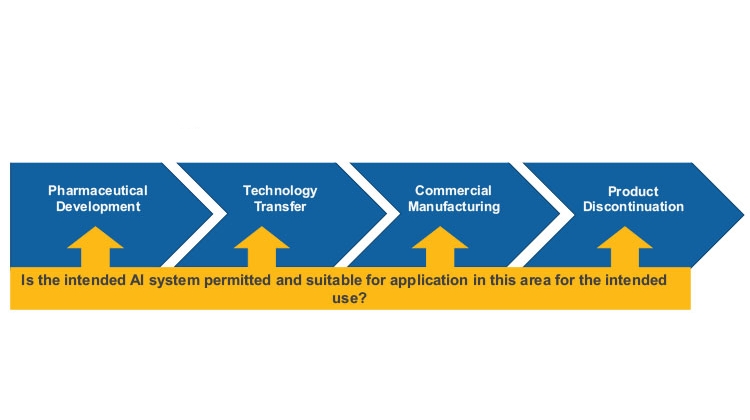
Artificial intelligence (AI) has the potential to benefit the pharmaceutical industry and its GxP-regulated areas. Several pharmaceutical companies are currently running digital pilots; 90% of large pharmaceutical companies have initiated AI projects.
The framework’s holistic concepts can be integrated with current regulatory developments that are driven by both international and national regulatory bodies.2, 3, 4, 5, 6
After having published the AI maturity model7 with regard to autonomy and control, including a dynamic development path along the life cycle of an AI application, we continue our article series with our AI governance and quality assurance framework. This framework provides a general organizational and procedural structure for developing and sustaining AI solutions in GxP-relevant contexts.
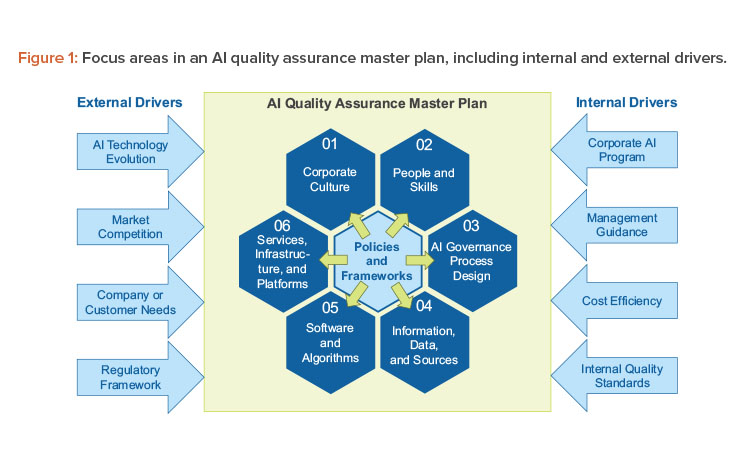
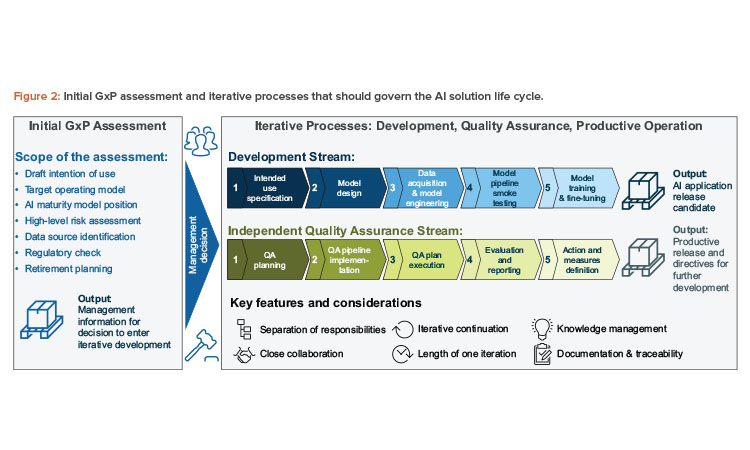
Our holistic concept covers the focus areas shown in Figure 1, packaged in an AI quality assurance master plan. This overarching structure enables harmonization across AI initiatives from a top-down approach while retaining the flexibility to tailor the operational procedures for each initiative that would be governed by this master plan and facilitates respective cooperation across AI initiatives:8
- Corporate culture: The development of AI solutions generally requires a shift in mindset by embracing change and adaptive learning on both the corporate and individual levels as opposed to “frozen state” approaches.
- People and skills: Effective AI development and quality assurance require a large set of stakeholders—typically organized in different business units—who need to be aligned in a structured manner to foster a collaborative environment.
- AI governance process design: AI solutions are inherently evolutionary in their nature. Their purpose is to continuously learn from new insights and data. Therefore, the process design must support this iterative nature and simultaneously ensure the quality required in a GxP-relevant context.
- Information, data, and sources: These assets are the fuel for every AI solution, and they need to be carefully evaluated with regard to quality standards.
- Software and algorithms: AI-featured algorithms come in many forms, from self-developed to freely available software. In addition to the choice of the actual AI model, the implementation is important to consider, in particular given the complex nature of many AI algorithms (e.g., deep neural networks).
- Services, infrastructure, and platforms: AI solutions are typically accompanied by large amounts of data. Real-time performance hardware and infrastructure are required for the AI solution to run during production.
This article covers (see Figure 2):
- Overview of the process design: In this section, we present an overview of the processes that should accompany the life cycle of an AI application.
- Initial GxP assessment phase: As a first step, we propose a structured preliminary analysis, which should assess whether an AI solution should be introduced in a specific context.
- Iterative process design: Reflecting the evolutionary nature of AI solutions, we propose a process design that develops iteratively. Our step-by-step approach includes quality assurance activities and clearly delineates responsibilities for all those involved in the process.
Overview of the Process Design
The AI governance process design begins by asking the following question: Where should AI be applied in the product life cycle so it leads to enhancements of the existing quality management system and ensures appropriate governance and risk management related to the application of AI in a regulated environment? To answer this question, consider that AI applications are evolutionary by their very nature:
- As new data are generated and collected, the AI solution should adapt to new situations or refine former results for continuous improvement.
- As technology evolves, and new AI algorithms become feasible, new modeling opportunities arise that may provide more value from a benefit or risk perspective.
- As AI solutions build incremental understanding for the use cases and the best modeling alternatives, new use cases might be
- identified in the course of the AI application’s life cycle.
- As the regulatory framework and interpretation changes, new requirements may be imposed that provide new opportunities for applying AI solutions.
With the interconnection of AI, existing quality management systems, and classical computerized systems in mind, the proposed high-level AI governance process design consists of three dedicated phases:
- Project initiation and initial GxP assessment should provide a valid entry point for the actual development of the solution, guided by a clear management decision.
- Development, quality assurance, and productive operation should be conducted via an iterative, yet tightly controlled, approach and reflect the evolutionary nature of AI solutions.
- Product discontinuation and retirement should be considered, even at the initiation of the project, especially in an AI context since the data characteristics—and therefore the results—may drastically change when the solution is phased out.
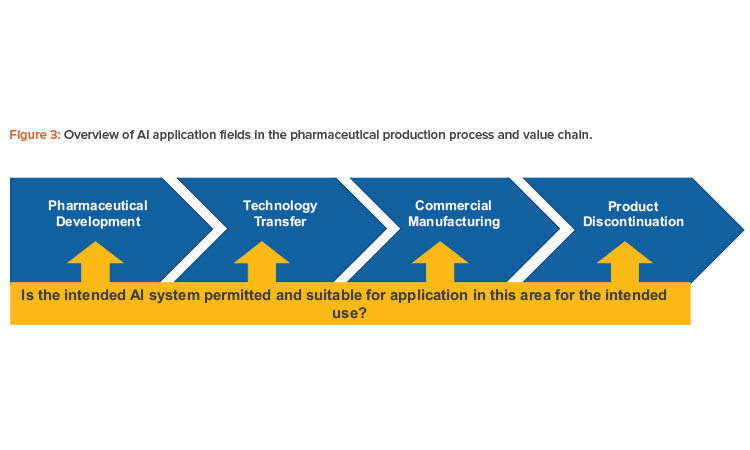
Initial GxP Assessment Phase
AI systems that will function in the GxP area, such as inspection systems in production or systems processing pharmacovigilance data, need to comply with the classical pharmaceutical models for a quality management system as proposed by the International Council for Harmonisation of Technical Requirements for Pharmaceuticals for Human Use (ICH) Harmonised Tripartite Guideline Q10: Pharmaceutical Quality System.7
This model for a pharmaceutical quality system can be implemented throughout the different stages of a product life cycle, from pharmaceutical development to technology transfer to commercial manufacturing, until product discontinuation (see Figure 3).
The elements of the pharmaceutical quality management system include the following: Process performance and product quality monitoring system; corrective action and preventive action (CAPA) system; change management system; and management review of process performance and product quality.
Since substantial resources may be involved in the development of an AI solution, an informed management decision should be made regarding the general feasibility of the AI solution. To facilitate the decision-making process, formal assessments for planned AI use cases supporting the quality management system elements within the life cycle phases should be implemented to answer the following key questions:
- Is the implementation of a planned AI use case permitted?
- Are there any external requirements (e.g., regulatory, ethical, legal, or customer related) that prohibit the use of AI?
- Are there any internal requirements (e.g., business sector, organizational) that prohibit the use of AI?
- Is an AI approach suitable for the specific use case?
- Is the impact on processes, functionality, and data integrity fully transparent?
- Are risk assessments, including acceptable risk mitigation measures, applicable?
- Can we expect data of sufficient quality (for development and during production) for the AI system to operate in production?
To answer these questions, the following are required: a draft of the intention of use, the operational design regarding human oversight, a high-level risk assessment, a regulatory check of whether an AI solution is actually permitted to be applied in this context, and the identification of suitable data sources.
All relevant stakeholders should be included in the assessment to consider all aspects of a planned AI use case; at a minimum, process owners (business), system owners (IT), and quality delegates should be represented in the evaluation. From a management point of view, suitable personnel should be identified who will be in charge of development, quality assurance, and productive operation. At this stage, the retirement approach of the AI solution should be drafted (“exit strategy”).
Iterative Process Design
As part of the iterative process design, we suggest two streams: one focusing on development activities, and the other focusing on stringent quality assurance. However, these two streams are closely interlinked and provide feedback as well as defined artifacts. At the same time, this design provides for the separation of duties to ensure a four-eye principle for the development of AI solutions in GxP-relevant contexts. In this case, four-eye principle means that any AI application may go productive only if at least two independent parties, as in the development and the QA stream, have assessed its quality. Further layers of control would be added with management involvement and potentially additional parties such as external auditors.
The separation of duties between the development stream and the independent quality assurance stream of the intended use can be achieved using one of the following means:
- Organizational: Separation of development and the involvement of independent quality assurance.
- Procedural: Separate responsibilities among development and quality assurance within an integrated process, but with different process owners in person.
These approaches should ensure that the quality dimensions that are required for safe and effective productive use are met. These concepts are summarized in Figure 4.
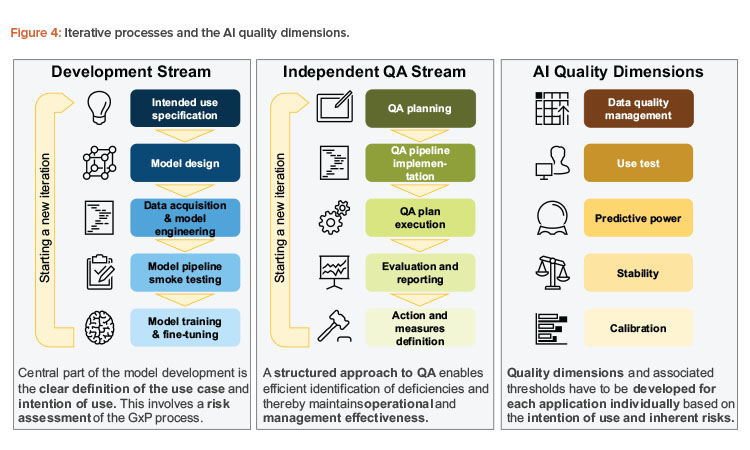
An iteration leads to a defined version of the AI solution and covers both the development and the quality assurance streams. An iteration may last as long as the use case requires. The following aspects should be considered:
- Longer iterations involve more risk for the current implementation phase and increase the potential for friction between the development and the independent quality assurance streams.
- The lengths of the iterations may change during the lifetime of the application as long as the two streams are appropriately synchronized.
- Relevant input for the length of the iteration should depend on the speed of new data and the input generated by customers, patients, or stakeholders, which originates from post-marketing surveillance activities.
Development Stream
The development cycle involves all activities needed to produce an AI release candidate, i.e., a packaged solution that can be deployed on a suitable infrastructure and that will be assessed for fitness for production along with required documentation. Multiple cycles could be applied during the life-time of the AI application, which means that there are two general types of development cycles:
- Initial development iteration: Usually, only historical data and a draft for the intention of use are available in the first development cycle. Also, the development should be completely decoupled from production in order to mitigate any risks on the actual GxP-relevant process.
- Subsequent iterations: Later development cycles profit from a more refined intention of use and risk assessment as a basis for further development. In addition, these cycles may react to findings generated during independent quality assurance and post-marketing monitoring in case a version of the AI system is already in operation. The development activities should be conducted in a manner that mitigates any risks on the actual productive process.
However, the following structure meets the needs of both the initial and subsequent cycles by following a five-step approach:
- Intended use specification: In the beginning of every cycle, it should be specified what optimization targets the AI solution should achieve. In addition, the specific environment (e.g., physical environment, users, and other stakeholders) in which the application will operate should be specified. The initial analysis is concluded by a stringent risk assessment regarding AI-specific risks and other risks related to the application. The intended use may be expanded or altered in each cycle while maintaining an overview of the application’s target and its inherent risks.
- Model design: Given the intended use, a suitable modeling strategy should be chosen from clustering analysis, binary decisions, or probability estimates. Suitable data sources and use-case-driven feature definitions may be created. With a use-case-driven approach, all techniques to design features from their expected behavior within the data set or the classifier without necessarily doing a quantitative analysis at this stage of the process are in place. Hence, the expert expectation is formulated, which is assessed and augmented based on the data-driven features in the following steps. As a result of this phase, a functional model specification is created that shows how the AI solution is designed to solve the problem imposed by the intention of use.
- Data acquisition and model engineering: This step involves all activities necessary to turn the model design into a working AI system in a development environment and potentially a test environment. These activities typically include the following:
- The provision, preparation, and quality assurance of selected data per the model design. Data might need to be augmented or imputed as justified by the use case.
- The implementation and packaging of the actual AI software and its adjacent non-AI components.
- The implementation of deployment routines that deliver the AI system to a suitable infrastructure.
- Model pipeline smoke testing: In this step, the model mechanics should be quality assured. Crucial points are data interfaces (e.g., input data or parameters) where the adherence to the data and model conventions should be checked (e.g., positive or negative weights). Furthermore, the non-AI elements of the solution should be verified using classical computerized software validation.
- Model training and fine-tuning: Once the model can be applied to the data, the model should be trained on a defined training set. Based on the first results, the model may be fine-tuned, and further features may be developed while reaching a set of suitable models for productive use and challenger models (i.e., models that are running parallel to the productive model to provide ideas for further improvements). In order to measure the improvement during fine-tuning, the development team will implement suitable quality measures to reach the optimum model given the intention of use. The result of this step is a set of potentially (i.e., from a technical point of view) releasable models, ready for subsequent quality assurance activities.
Independent Quality Assurance Stream
The independent quality assurance stream should be applied as often as the development stream runs. With potential additional runs (e.g., for regular or ad hoc quality inspection), this process should be streamlined as much as possible. The five-step approach mimics the development cycle:
QA planning
The scope of analysis—based on the intention of use and identified risks—should be determined, involving acceptable qualitative and quantitative outcomes and measures. In addition, specific action should be formulated if thresholds or limits are not met as guidance for the further development of the AI solution.
QA pipeline implementation
Since the quality assurance should be run often in this iterative setting, analyses and quality assurance steps should be automated to the extent possible. Although most of the quality assurance activities should be automated, a process may start by relying more on manual steps if the integrity of the quality assurance outcomes are protected. This quality assurance pipeline should be tested with regards to good software development practices, including performance summaries and management reports. Finally, more organizational and qualitative facets of the quality assurance exercise should be aligned (e.g., subject matter expert or user interviews and expert panels) to allow for a smooth operational process.
AI systems that will function in the GxP area need to comply with the classical pharmaceutical models for a quality management system.
QA plan execution
Once the AI application’s release candidate is handed over from the development stream to the independent QA stream, the release candidate is deployed on the QA team’s infrastructure and suitable test data is delivered to their environment. The QA team is, in general, responsible for the test data that is delivered, especially with regard to the representativeness of the data vis-a-vis the intention of use. However, as the provisioning of test data may require complex data pipelines, the QA team may leverage existing data pipelines that were developed during the development stream as long as they retain full responsibility for the delivered data. Now, the quantitative quality assurance analysis is executed. Furthermore, ad hoc and qualitative analyses are conducted, and the results collected. An important aspect of these exercises is a traceable environment to allow for a post-marketing audit; in particular, all quality assurance results need to be reproducible in reasonable time. The time frame in which the results may be reproduced may vary with the use case. As a general guidance, the timespan of the original QA exercise runtime plus an additional setup time up to several days should be justifiable. In our view, more important is the exact reproducibility of the results that were obtained at the original run rather than the time to retrieve the replica.
Evaluation and reporting
The quality assurance results are investigated, and potential deficiencies are identified given the thresholds and targets in the first step. The results are prepared for high-level decision-making, which involves management recommendations and actionable measures, ranging from the deployment decision of the release candidate to specific areas of improvement as guidance for the next implementation cycle.
Action and measures definition
On an appropriate management level, a decision is made whether to continue with the AI solution. Crucial input for those decisions involves the quality assurance results and the functionality-oriented intention of use and risk assessment. The action definition may involve adjustments to the quality assurance framework itself (e.g., measures and quality assurance approach or thresholds). Although actions guide the further development of the AI solution, measures are designed to mitigate risks that may be identified during the development and quality assurance of the model, potentially based on post-marketing information.
A particularly important aspect in the context of GxP is CAPAs. Because CAPAs focus on clear deficiencies of the release candidate under investigation, measures of this kind should have priority against the continuous improvement of the model. CAPAs may be defined based on deviations in the overall quality assurance outcomes of the model (e.g., its predictability or any potential in bias) or from available single incidents reported via post-marketing studies or other post-marketing information.
Intention of Use, Risk Assessment, and AI Quality Dimensions
The core of each AI application is the intention of use (i.e., what the application should achieve). By safeguarding the application of the solution, a risk assessment identifies potential risks before release and directs the development and quality assurance activities to mitigate those risks while providing the benefits specified in the intention of use. In the following subsections, we show how these items are interlinked and illustrate the application with specific examples in GxP-relevant contexts.
The following overview shows how the intention of use specification, the AI-specific risk assessment, and quality dimensions can be identified in a structured manner and what can be concluded from these steps. These activities, as well as the actual monitoring of the performance metrics themselves, should be seen as an ongoing process, since new signals originating from post-marketing surveillance or follow-up studies after adopted in pro-duction may shift the AI application’s intention of use, the risk profile, and the quality measurement. Also, this analysis may provide input for the positioning in the maturity space for the target operating control model design of the AI system.
The intention of use should clearly communicate the purpose of the AI solution:
- What the application should achieve and in which environment the application should operate (physical environment, users, patient groups, and other stakeholders).
- What alternatives exist and why an AI solution might provide additional benefits.
- The AI-specific risk assessment should reflect the stochastic nature of the AI application in addition to classical risks:
- What physical, legal, or budgetary impact might arise from misclassifications or inaccurate results to the patient, the user, the organization, or others? How much would this distort acceptance and trust in the data and solution?
- What risks might threaten the AI development and quality assurance iteration or stream as a whole?
- Quality dimensions should be tailored to the AI solution such that identified risks are effectively and communicably monitored; and suitable thresholds are defined that capture the state-of-the-art expectations to the AI solutions outcomes and alternative means for fulfilling the intended use (if available).
- Measures should be defined based on the risk assessment to mitigate the risks that were identified in the risk assessment or given the outcomes of the quality dimensions. Measures should be proportionate with regard to the risks involved and the human oversight involved in the operation of the AI solution; the choice of human control as a mitigation strategy is an important factor to shield against AI errors and to foster trust into the application of the AI solution. A clear rationale—qualitative and/or quantitative—should be provided that shows the suitability of said measures, focusing on risk mitigation.
The regular evaluation as per the quality assurance stream should provide a decision basis for the subsequent development activities and measures. Regarding the release of a new version, an AI solution release candidate passes the quality assurance check if risks are mitigated according to the quality dimension standards, and if it can be demonstrated that the model is the best choice given the current state-of-the-art data, development, and quality assurance.
The choice of measures, rigor, and transparency implemented depends on the risk assessment of the AI application. The same risk assessment methodology should be applied for all AI systems within the corporation and follow defined, clear, and sensible criteria leveraging already implemented risk assessment processes. The impact on risk toward patient/consumer safety, product quality, and data integrity will drive the quality assurance of the AI system and regulatory burden. It should be noted that from a regulator’s perspective, a risk-based approach is also desired, and inspections focus on critical systems with an impact on public health. An established strategy is the two-stage risk assessment that involves (a) an initial risk assessment and determination of the system impact (GxP applicability determination) and (b) functional risk assessment on the user requirements and system functionality as described in the introductory part of the AI governance process design.
To provide a structure for measuring the AI application’s performance with respect to the intention of use and the risk assessment, five quality dimensions can be used to validate the stochastic nature of AI applications:
- Data quality management: Does the productive data adhere to data expectations? Is the data in the training set representative of productive use?
- Use test: Has the system been used according to its intention, for its target group or target operation, and according to the specified user–machine interaction?
- Predictive power: Has the system been able to effectively predict the desired outcome based on its input?
- Stability and robustness: Does the model provide consistent outputs with regard to the evolution in time of input data and the model itself?
- Calibration: Does the model exhibit biases on a global level or for particular, undesired stratifications?
Although all quality dimensions are relevant to AI applications in general, the actual focus and selected measures can be tailored to the intention of use and the risk assessment. This means that measures and thresholds of quality dimensions should be chosen in a risk-based manner, reflecting the most critical aspects of the AI solution as per risk assessment. Also, priorities and trade-offs have to be chosen in this regard; for example, the predictive power and the stability commonly result in conflicts that have to be resolved based on stakeholder (i.e., users, patients) expectations and the risk appetite in line with the corporation’s AI strategy.
The AI governance and quality assurance framework ensures both full and auditable process control and agility.
Conclusion
While AI- and machine-learning-specific regulations are currently under development, more detailed guidance is needed to turn these regulations into AI solutions that can be applied in GxP-relevant contexts. With its stepwise process design, the AI governance and quality assurance framework ensures both full and auditable process control and agility, which are necessary to successfully benefit from these new technologies and unlock their full potential. Specific tasks and responsibilities are encapsulated in a structured manner but are still flexible enough to be applied to a specific context of an AI solution. In further publications, we will elaborate on other focus areas of our AI governance and quality assurance framework, with further details regarding both technical considerations (e.g., IT security) and organizational challenges when introducing AI development at a corporation. We believe that the approach described in this article has considerable potential for application in other life science industries.
About the Authors
Acknowledgements
The authors thank the ISPE GAMP® D/A/CH Working Group on AI Validation, led by Robert Hahnraths and Joerg Stueben, for their assistance in the preparation of this article, and Markus Kaufmann and Rolf Blumenthal for their review.